Project lead: Youngjun Choe, Ph.D., Assistant Professor, University of Washington, Industrial & Systems Engineering
Data scientist lead: Valentina Staneva
DSSG fellows: Sean Andrew Chen, Andrew Escay, Christopher Haberland, Tessa Schneider, An Yan
Project Summary: When a hurricane makes landfall, situational awareness is one of the most critical needs emergency managers face before they can respond to the event. To assess the situation and damage, the current practice largely relies on driving around the impacted area (also known as a windshield survey) by emergency response crews and volunteers. Recently, drone-based aerial images and satellite images have helped improve situational awareness, but the process still relies on human visual inspection. These current approaches are generally time-consuming and/or unreliable during an evolving disaster.
The governing research question of the project is: Can a machine learning algorithm automatically annotate damage on post-hurricane satellite images? To answer the question, the project uses satellite imagery data on the Greater Houston area before and after Hurricane Harvey in 2017, and damage labels created by crowdsourcing. If the project results in a successful algorithm (which is trained to quickly detect ‘Flooded / Damaged Building’, ‘Flooded / Blocked Road’, and ‘Blocked Bridge’ on a satellite image for a new event), it will be an exciting technological innovation to improve situational awareness during the first response to hurricane-induced disasters.
Project Outcomes: The team successfully developed an algorithm that automatically creates bounding boxes around flooded/damaged buildings with a mean average precision of 0.47 on a testing dataset of the satellite images. This achievement was possible because the team found and processed auxiliary datasets (e.g., building footprints created by the Oak Ridge National Laboratory and Microsoft) to create appropriate training datasets for object detection models. Furthermore, the team found aerial imagery of the Greater Houston area taken after Hurricane Harvey by the National Oceanic and Atmospheric Administration, and extensive damage labels collected by the Federal Emergency Management Agency. These additional datasets enabled the team to develop another algorithm to detect flooded/damaged buildings with a mean average precision of 0.32 on a testing dataset of the aerial images. Building upon the project outcomes, the Disaster Data Science Lab will continue the research.
View the final presentation slide deck (PDF). Watch the video on YouTube. Related links: Github repo. View the project poster presented by Christopher Haberland at the Cascadia Innovation Corridor Conference in Vancouver, B.C. in October 2018.
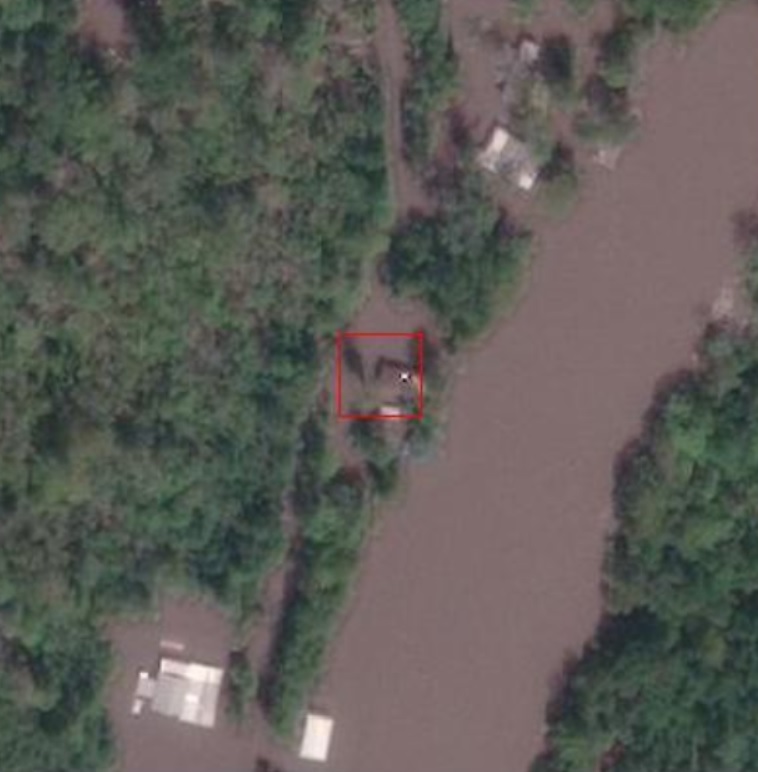
Blog posts:
A quicker recipe by Chris Haberland
Reflections on reproducibility of data science projects by An Yan
Getting ground truth data through geospatial analysis by Sean Chen
Is garbage in always garbage out? by Andrew Escay
Machine learning can improve disaster response after hurricanes, but not alone by Tessa Schneider