Project leads: Tim Althoff, Assistant Professor, Computer Science & Engineering, University of Washington; and Dave Atkins, Research Professor, Psychiatry and Behavioral Sciences, University of Washington
Data science lead: Valentina Staneva
DSSG fellows: Shweta Chopra, David Nathan Lang, Kelly McMeekin
Project Summary: Everyone encounters challenges in life – whether that includes stress from school or work or more significant problems like depression and addiction. When we hit these challenges, we often reach out to friends and family for emotional support and problem-solving help. Peer support is an extension of this and has a long and well-researched history as a first line of intervention for mental health and addiction problems. Traditionally, peer support was in-person, but technology advances mean that support can be ‘crowdsourced’ and scaffolded and thus taken to scale.
However, by its nature, peers are not licensed counselors, and thus, it is critical to provide feedback on what really works and is helpful vs. what might be very well-intentioned… but not so helpful. Our Data Science for the Social Good project will focus on using data from an online peer support platform to better understand what types of responses are the most helpful to young adults sharing their struggles online. We will pursue this objective by analyzing a large-scale dataset of around 100 million posts and interactions. We then want to use these insights to develop tools and trainings for peers to help them be as helpful as they can when supporting others in need.
Project Outcomes: The Peer Support team met a number of key objectives during our 2019 DSSG project. First, our team used data from a real-world, online peer support platform, including over 20 million posts and responses. A key initial task was accessing, comprehending, and filtering this large amount of data. Specifically, we developed a series of selection rules to narrow down the data to focus on posts and responses dealing with mental health and related concerns.
A second task relied on natural language processing (NLP) tools for summarizing large text corpora to answer the foundational question: What are the posts and responses focused on? What are people on the platform requesting help for? Using latent dirichlet allocation (also called, topic models), our team summarized major content themes, revealing that expressions of hopelessness and despair were very prevalent, as were encouragements in response.
A third important task was using social media like indicators within the platform to identify and evaluate markers and proxies of helpful behavior. There were no ground truth assessments of helpfulness, and thus, we used indicators from the platform such as self-reported changes in mood, “likes”, and expressions of gratitude derived from the text of posts and responses.
Finally, our team used machine learning and NLP models to predict helpfulness indicators using text-based and derived features. The text-based features in particular begin to describe what helpful responses look like in an online peer support environment. A summary of our work and outcomes was provided to our key stakeholders, and future work will continue to develop how NLP-based methods can help inform maximizing the help of online peer support platforms.
Learn more by viewing the final presentation slides, project website and project blog. View a video of all four final presentations here.
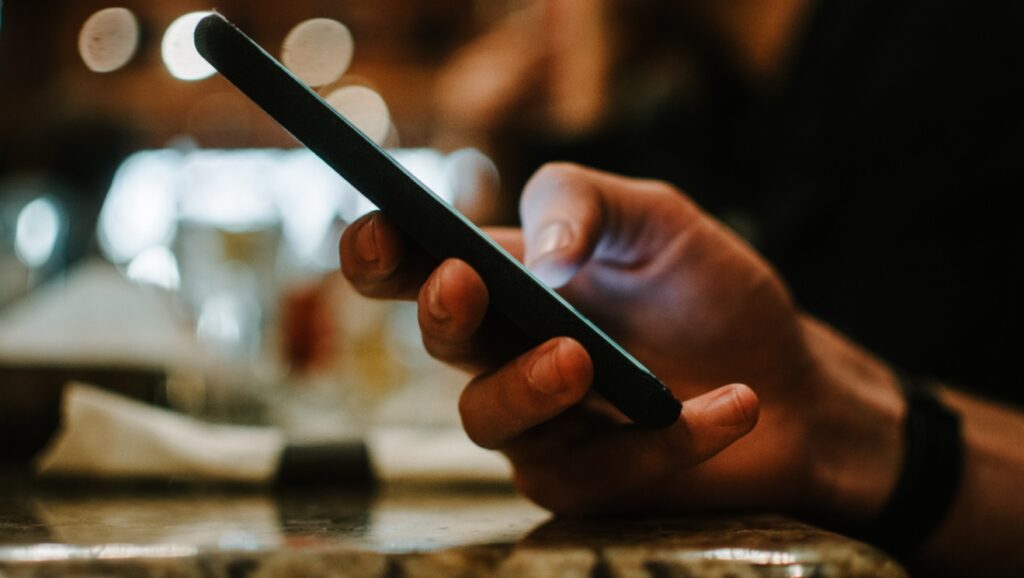