August 14, 2019
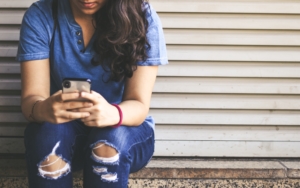
Young adults who face mental health struggles often turn to social media for peer support, leaving a large data trail in a field that has been traditionally documented through qualitative work. A team at the eScience Institute’s Data Science for Social Good (DSSG) program at the University of Washington (UW) is conducting an analysis of these interactions to understand what types of posts and responses are the most helpful to those who are suffering.
The project examines a large online peer support network, where the typical user is 15 – 24 years old and facing depression, anxiety, self-harm, or suicidal thoughts. Kelly McMeekin, a database management student at South Puget Sound Community College and one of three student fellows working on the project, summarized the research questions as: “What does it mean to be helpful? How do we measure that? How do we predict how helpful a response will be?”
To answer these questions, the team is using Dask, SQL, Python, and logistic regression modeling on a dataset totaling 5.4 million rows of online posts and comments pulled from an original database of over 200 million rows that they accessed through a data license. The platform has about 500,000 users. Interactive features include the option to “like” a post, comment in a thread, follow people, and express other emotional reactions to posted content. When creating a post, users can select a mood and topical category, such as family, health, or bullying.
“We’re hoping just through mining and filtering these data, we might be able to identify a set of behavioral moves and nudges that encourage more thoughtfulness and helpfulness. If a person is really hurting, this might offer a form of in-the-moment support,” said fellow David Nathan Lang, a Ph.D. candidate at Stanford University’s Graduate School of Education.
The data presents challenges. The platform represents a mix of social media and peer support content, and parsing the two can be complex. Another complication is that there is a median of one interaction per participant, which makes it difficult to track communication patterns or detect the meaning of a particular post, yet filtering out infrequent users would create a bias in the dataset. The vast size of the dataset can slow processing, although creating subsets of data to work with has helped alleviate this challenge. The team recently discovered one outlier post with 28,000 comments – compared to an average of around 10 comments per post – which had swayed some of their data analysis.
Valentina Staneva, the project’s data science lead and a senior data scientist at the eScience Institute, said the overarching research questions help constrain the team’s work and avoid erroneous conclusions that can result from analyzing a huge dataset. “In a big data set, you can always deviate and find something. But it might be just spurious correlations and you start making conclusions. It makes you feel like you have made a discovery. Having an underlying question reminds us: what is our objective?” she said.
The two project leads are Tim Althoff, assistant professor in Computer Science & Engineering, and Dave Atkins, research professor in Psychiatry and Behavioral Sciences, both at UW. “Even if you’re somewhat versed in what this might feel like, even if you’re motivated to help somebody, it’s still pretty hard to have the right words in these moments,” said Tim, describing the significance of the project. “What you’re currently presented with when you go to one of these sites is an empty text box, and volunteer peers try to help each other, but it’s a complicated messy process that we don’t understand very well.”
Without the ability to get feedback directly from users about what they found helpful, the team has had to come up with other potential indicators of helpfulness such as liking a post, thanking a commenter, or logging an improved mood after a negative one. However, the team acknowledges that these substitute “helpfulness” measures are problematic indicators due to variable usage patterns that result from social norms and social media behavior. The team is applying natural language processing (NLP) methods to the online interactions to determine which factors have the strongest correlation to “helpfulness.”
The text and NLP methods of analysis include the bag-of-words model that breaks the text down into a set of multiple words. This enables the team to find which words correspond more with the notions of helpfulness that they have defined. The team is also generating additional analytical features by tagging posts that reflect traditional counseling methods. This part of the study is based on motivational interviewing techniques used by counselors, which group statements into categories such as “reflections” or “affirmations.” The team has also taken user-specific features like age, length of time on the platform, and posting frequency, into consideration.
Fellow Shweta Chopra, a master’s student in Social Policy and Data Analytics at the University of Pennsylvania, said the project has taught her the importance of thinking through atypical scenarios known in engineering as “corner cases” before starting an analysis, particularly when working with data of this scale. The use of parallelization techniques to speed up processing tasks has also been a significant learning experience.
Team members are currently running models and identifying patterns in a 2018 sub-sample of the data as part of the interpretation stage of the project, during which time Shweta said, “We will pore over the results the models are giving us. How can we improve them and what kind of insights can we derive?” The team members are also working to clearly identify assumptions and methods in their analytical processes to provide transparency about steps taken, such as filtering out social media content that is intermingled with peer counseling.
The knowledge gained through the project will be relayed back to the peer support platform for consideration as they look to improve user-commenter interactions, and the team hopes that their project will inspire more rigorous research and analysis of peer support platforms moving forward.
Final Presentations by all four of this year’s DSSG project teams will take place from 3 – 5 p.m. Wednesday, Aug. 21. The event is open to the public.