Nineteen project leads, eScience data scientists, and researchers participated in this year’s Winter Incubator program, which focused on projects in hydrology, political science, astronomy, atmospheric science and neuroscience. The five teams met over the course of 10 weeks to develop their projects, and presented their results on Mar. 13, 2018 in the WRF Data Science Studio.
Details on the projects can be found on the program’s GitHub page. The next opportunity to participate in the program will be in 2019 and a call for proposals will be announced this fall.
As we did last year, we offered project leads the opportunity to give feedback on this program. Here are the responses we received.
Qiaoyun Peng, Department of Atmospheric Sciences
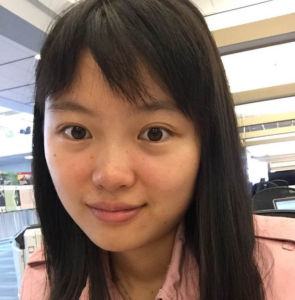
The Incubator program was a very special learning opportunity to me. As a graduate student in Atmospheric Sciences, I deal with various trace gas fluxes to figure out their source and sink functions as well as impact on air pollution and climate. I was curious about the drivers behind the flux dynamics but couldn’t answer it with traditional surface models due to its lack of robustness in capturing complex nonlinear characteristics.
Inspired by the emerging data-driven methods, I applied to the Incubator program hoping to simulate the gas exchange dynamics using mechanism-free machine learning approaches. It turned out to be fruitful – the present study validated the feasibility of synthetically estimating flux with ML techniques and it showed statistically significant results. This interdisciplinary experience enlightened me to incorporate more data-driven methods in my future research.
Moreover, I think the Incubator get-together every week is very helpful. Meeting and talking with peers and mentors gives me valuable feedback and direction on what I am working on. This is way better than just sitting in front of computer myself debugging through flux codes. It also gives me a chance to learn about the fellow incubatees’ work and share our research experience. In a word, I am really grateful to be an incubatee and look forward to further collaboration with scientists at the eScience Institute.
________________________
Chad Curtis, PhD student
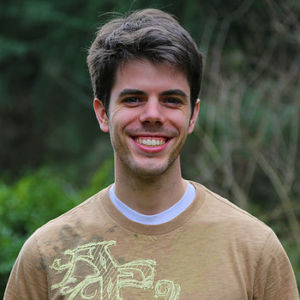
I had the great opportunity of working side by side with Dr. Ariel Rokem at the 2018 Winter Incubator, and I couldn’t be more enthusiastic! I am a third-year chemical engineering student examining the interactions of nanoparticles with the brain microenvironment. A key analysis tool central to our lab is multi-particle tracking. This allows us to extract information on the diffusive characteristics of nanoparticles from high-speed video collected with fluorescent microscopy.
However, while there are many tracking analysis packages out there, there is a lack of information regarding the practical problem of scaling up the tracking process; we will often need to analyze thousands of videos at a time, which would be insurmountable if we had to analyze each video individually. This was one key problem I brought to the table to this year’s Incubator.
Through my experience in the Incubator, I was able to greatly increase the efficiency of my current workflow by parallelizing my tracking analysis using Amazon Web Services. With the help of expert staff at the eScience Institute, I was also able to solve our long-term data storage problems.
We can generate multiple terabytes of video in a month, which we had previously been storing on individual hard drives. Now we are implementing a two-step backup protocol using S3 and Glacier. In addition, we greatly increased the amount of information we are able to capture from our tracking analysis. We added a features analysis module that is able to extract and visualize geometric parameters associated with each trajectory in a video.
Coming into the Winter Incubator, I had previous coding experience in Python, R, and Matlab. However, my knowledge was limited to solving the whatever immediate technical analysis problem was at hand. During the Incubator, I learned how to work in a collaborative setting using GitHub, implement real-time testing of code, bundle my code into a shareable package format, and create high-quality documentation for my code. The Incubator ultimately helped lessen the mystery of data science and helped me feel a part of a community that had previously seemed to speak a different language.
I left the Incubator feeling much more independent as a data scientist, and with many more questions based off my work during the past nine weeks. I am grateful to this awesome program, and I look forward to keeping the momentum going!
________________________
Christina Bandaragoda, senior research scientist, Watershed Dynamics Research Group, Civil & Environmental Engineering
Snow and ice are critical natural reservoirs of water resources. Improved understanding is expected to improve management decisions, planning, climate impact assessment, flood and drought resiliency. Before the Incubator, hydrologic modeling predications were reported with unknown uncertainty estimates.
The work accomplished during the Winter Incubator resulted in a standard method for ensuring ergodic, optimal model behavior required to support hypothesis testing used in scientific decision making. Given the hours of model run time required, the computing demand could not have been possible without the help of UW IT Cloud Services and Amazon Web Services.