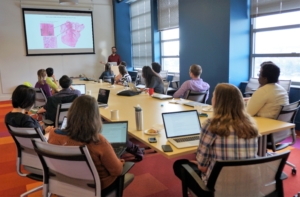
By Robin Brooks
For ten weeks over the winter 2017 quarter, the UW eScience Institute hosted its annual Winter Incubator Program, a collaborative intensive designed to connect data scientists from the Institute and domain scientists with the goal of fostering new research discoveries. Projects were selected from a pool of applications, and the work began in January.
Research topics varied, and participants utilized data science techniques such as data visualization, machine learning, and image processing to further their work.
Aaron Marburg and Wu-Jung Lee of UW’s Applied Physics Laboratory led projects in oceanography research, while Catherine Kuhn of the School of Environmental and Forest Sciences worked on a freshwater monitoring tool.
Alicia Clark (Mechanical Engineering Department) focused on particle tracking in low-contrast images. Dr. Nicholas Reder, UW Medicine Pathology, worked on a light-sheet microscopy challenge, and Owen Williams (Department of Aeronautics and Astronautics) used machine learning techniques to study turbulence.
The next iteration of the Winter Incubator program will occur in the winter of 2018; a call for proposals will go up on our website this fall.
We offered an opportunity to provide feedback on the program, and Dr. Reder and Wu-Jung Lee replied; their comments have been lightly edited for clarity and the hyperlinks were added.
____________
Nicholas Reder
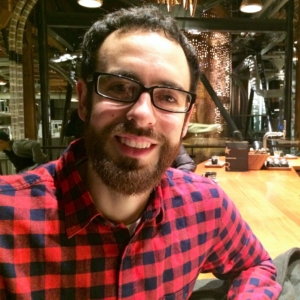
I participated in the UW eScience Institute’s Winter 2017 Incubator and would like to share my productive and rewarding experience with the program. I am a 3rd year pathology resident at the UW Medical Center who is working with a team of optical engineers to build a “light-sheet microscope” for improving the practice of pathology.
Pathologists examine tissue microscopically to make a diagnosis. Our current method is a century-old technique using thin sections of tissue on glass slides and a light microscope. Our lab built a light-sheet fluorescence microscope that produces high-resolution 3D microscopic images without the need to produce a glass slide.
This innovation is a major advance for the field of pathology, with benefits including faster turnaround time, more accurate diagnosis, and new biological insights revealed by examination of 3D microscopy data. There is one catch: the imaging datasets are massive and we cannot use existing software tools for image processing or visualization. Before the incubator, our lab group lacked the expertise to write new software to address these challenging big data problems. Thus, we used tedious and inefficient methods to process and visualize the data, and we stored the data on multiple external hard drives.
Through my experience in the Winter 2017 Incubator, our laboratory group now leverages cloud-based storage and computing to efficiently process, store, and visualize our imaging datasets. These new software tools increased the efficiency of our lab and we can now produce striking 3D visualizations of our microscopic imaging data.
In addition to the benefits to the lab, I have seen great personal benefits as well. Working side-by-side with an expert in data science (Dr. Ariel Rokem), I learned basic programming skills and data science fundamentals. The eScience WRF Data Science Studio provided a total data science immersion for two days a week. I had frequent conversations with data science experts and fellow Incubator participants sharing knowledge on a wide range of topics in data science. Now I can write my software, utilize best practices in development and documentation, and solve most problems myself rather than hitting a wall.
In summary, the Winter 2017 Incubator could not have been a better experience. Not only did our lab find a solution to our specific big data problems, we also learned the fundamentals of data science to implement in future projects. On a personal level, the incubator provided a unique environment to cultivate my interests in data science and boost my academic career. Thank you for this opportunity and I hope many budding data scientists get to share this experience in the future as well.
____________
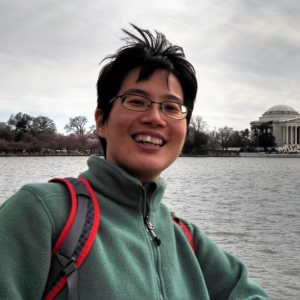
Wu-Jung Lee
The Winter Incubator program was an exciting and rewarding learning experience for me. This program helped me take a first crack on a project I have wanted to do for a couple of years – using machine learning approaches to analyze long-term sonar time series from ocean observatories. The goal of the analysis is to quantify the distribution dynamics of marine organisms across multiple trophic levels.
The Incubator setup, which involved teaming up with both data scientists and fellow “incubatees” by simply sitting together two days a week, made it very natural to exchange ideas and operational knowledge about a variety of problems. During the Incubator period, we compiled data from two cabled nodes in the Endurance array in the Ocean Observatories Initiative (OOI), and applied matrix decomposition methods to discover recurrent and emerging daily patterns in the large and complex sonar time series.
This lays the groundwork for further refinement of the methods to incorporate temporal continuity and correlation with oceanographic phenomena, such as mesoscale eddies, into the formulation for ecologically meaningful interpretation. Thanks to the Incubator, I am now able to actively pursue extramural funding sources with concrete preliminary results and a clear outlook for future milestones. I am excited about opportunities to continue my collaboration with scientists at the eScience Institute on data-intensive research in ocean sciences.