Project leads: Erin Trochim, Research Assistant Professor, Alaska Center for Energy and Power, University of Alaska Fairbanks
Data scientist: Nicholas Bolten
DSSG fellows: Vidisha Chowdhury, Maddie Gaumer, Philippe Schicker, Shamsi Soltani
Alaska– and the wider Arctic region– have experienced accelerated effects of climate change over the past decade. Rapid decarbonization of energy is a critical step for mitigating climate change and ensuring sustainable development. Since about 70% of energy consumption in Alaska can be attributed to commercial and residential heating, successful decarbonization requires an assessment of present and future heating needs in the region.
To date, comprehensive and accurate heating load estimates are lacking in Alaska. Modeling heating loads in Alaska is particularly complex for two distinct reasons:
- First, many communities do not connect to the Energy Railbelt utility grid, making it difficult to accurately measure their heating loads.
- Secondly, Alaska lacks centralization in utility providers and building codes, meaning there are no consolidated energy use data or efficiency standards for buildings.
This project develops a geospatial-first methodology using machine learning to estimate heating loads. It represents the first attempt to quantify Alaskan heating loads at scale and high granularity. We utilize open-access datasets and Google Earth Engine’s cloud computing platform to extract building features (e.g., height, square footage, and age) and local climate variables (heating and cooling degree days). We explore statistical and machine learning models and validate them against existing small-scale regional models from AK Warm simulation software.
This project has strong potential for scalability across the wider Arctic, which mirrors many of the energy security and decarbonization challenges faced in Alaska. Therefore, the models developed through this work offer a starting point for pan-Arctic energy estimation and climate change mitigation methods.
Learn more on the project blog and project website. Watch the presentation here, or the full recording of all four team presentations here.
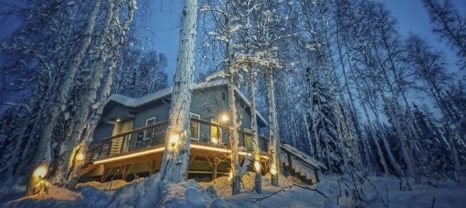
Project Presentations
Vidisha Chowdhury, Madelyn Gaumer, and Philippe Schicker presented the project at the Learning & Doing Data for Good (LDDG) conference at the University of Washington in September 2022.
Madelyn Gaumer gave the presentation: “Estimating Heating Loads in Alaska using Remote Sensing and Machine Learning Methods” at the Neural Information Process Systems (NeurIPS) conference in December 2022. This virtual poster presentation was part of the Workshop: Tackling Climate Change with Machine Learning.
Three fellows also made presentations at the American Geophysical Union Annual Meeting, which took place virtually in December 2022:
Philippe Schicker presented “Environmental Justice Considerations for Remote Sensing Approaches: Calculating Heating Loads in Alaska through Geospatial and Machine Learning Techniques.” This presentation was part of the SY44C: Critical Remote Sensing I Online Poster Discussion session.
Shamsi Soltani presented “A Novel Geospatial-First Machine Learning Approach to Modeling Heating Loads in Alaska: When Perfect (Data) are the Enemy of Good (Data).” This presentation was part of the GH23B: Statistical Methods, Machine Learning, and Big Data in Geohealth II Oral session.
Vidisha Chowdhury made the presentation: “Informing Decarbonization through Machine Learning (ML): A Geospatial ML Pipeline for Estimating Heating Loads.” This poster presentation was part of the “Addressing environmental challenges and sustainable development through Earth science applications utilizing Machine Learning” session.