Project lead: Mike Katell, PhD Candidate, UW Information School
Data science lead: Bernease Herman
Faculty Advisor: Peaks Krafft, Senior Research Fellow, Oxford Internet Institute
Community Engagement Lead: Meg Young, PhD Candidate, University of Washington Information School
DSSG fellows: Corinne Bintz, Vivian Guetler, Daniella Raz, Aaron Tam
Project Summary: In partnership with ACLU of Washington, fellows will create an Algorithmic Equity Toolkit—a set of tools for identifying and auditing public sector algorithmic systems, especially automated decision-making and predictive technologies. Extensive evidence demonstrates harms across highly varied applications of machine learning; for instance, automated bail decisions reflect systemic racial bias, facial recognition accuracy rates are lowest for women and people of color, and algorithmically supported hiring decisions show evidence of gender bias. Federal, state, and local policy does not adequately address these risks, even as governments adopt new technologies. The toolkit will include both technical and policy components: (1) an interactive tool that illustrates the relationship between how machine learning models are trained and adverse social impacts; (2) a technical questionnaire for policymakers and non-experts to identify algorithmic systems and their attributes; and (3) a stepwise evaluation procedure for surfacing the social context of a given system, its technical failure modes (i.e., potential for not working correctly, such as false positives), and its social failure modes (i.e. its potential for discrimination when working correctly). The toolkit will be designed to serve (i) employees in state and local government seeking to surface the potential for algorithmic bias in publicly operated systems and (ii) members of advocacy and grassroots organizations concerned with the social justice implications of public sector technology.
In this project, fellows will gain hands-on experience using and auditing machine learning algorithms in surveillance contexts, with a focus on understanding the inner workings of these algorithms. To center the perspectives of those directly affected by such systems, the team will also engage community partners’ input on the Algorithmic Equity Toolkit throughout its development.
This project touches on issues and methodologies relevant to a wide range of fields, such as law, public policy, sociology, anthropology, design, social justice, data science, programming, artificial intelligence, geography, mathematics, statistics, critical gender and race studies, history, urban studies, and philosophy.
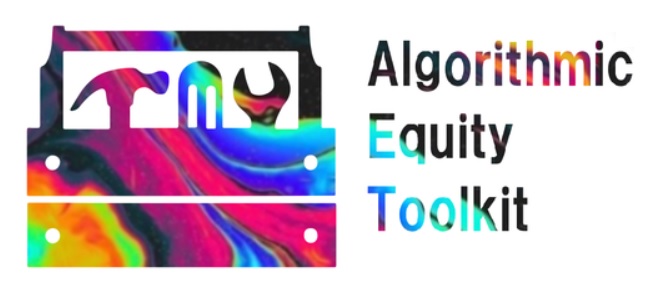
Project Outcomes: We iteratively developed our toolkit through a participatory design process that engaged data science experts, community partners, and policy advocates, while also drawing upon an array of prior literature and similar toolkit efforts. Initially, based on the regulatory focus of prior academic research, we envisioned that the primary users of the Algorithmic Equity Toolkit would be employees in state and local government seeking to surface the potential for algorithmic bias in existing systems. We thought advocacy and grassroots organizations could also find the toolkit useful for understanding the social justice implications of public sector systems.
Through our participatory design process, we refined our audience and design goals to focus on helping civil rights advocates and community activists—rather than state employees—identify and audit algorithmic systems embedded in public-sector technology, including surveillance technology. We achieve this goal through three toolkit components: (1) A flowchart designed for lay users for identifying algorithmic systems and their functions; (2) A Question Asking Tool (QAT) for surfacing the key issues of social and political concern for a given system. These tools together reveal a system’s technical failure modes (i.e., potential for not working correctly, such as false positives), and its social failure modes (i.e. its potential for discrimination when working correctly); and (3) An interactive web tool that illustrates the underlying mechanics of facial recognition systems, such as the relationship between how models are trained and adverse social impacts. In creating our own toolkit, we followed a weekly prototyping schedule interspersed with stakeholder feedback and co-design sessions.
The underlying questions that drove our design of these components were: What ethical issues should civil rights advocates be concerned with in regards to surveillance and automated decision systems? How are algorithmic systems reinforcing bias and discrimination? What do community organizers and non-tech experts understand about algorithmic tools and their impacts? What should they know about surveillance and automated decision systems to identify them and know how they work? In comparison to existing resources, which tend to target software engineers and in some cases policymakers as an audience, we focused on policy advocates and community activists as users.
Learn more by viewing the final presentation slides, project website. and project blog. View a video of all four final presentations here. Read the paper, Toward Situated Interventions for Algorithmic Equity: Lessons from the Field, presented at the January 2020 Association for Computing Machinery (ACM) Conference on Fairness, Accountability, and Transparency.
ACLU Washington published the Algorithmic Equity Toolkit in June 2020. Program participants are among the key contributors listed on the ACLU-WA website.