Project lead: Mark Hallenbeck, senior data science fellow, director of the Washington State Transportation Center
Project mentor: Michael Wolf
Data scientist leads: Jake VanderPlas (primary), Bryna Hazelton (secondary)
DSSG fellows: Mayuree Binjolkar, Daniel Dylewsky, Andrew Ju, Wenhao Zhang
Project Summary: Seven regional transportation agencies in the greater Puget Sound region use a common electronic fare payment system, called One Regional Card for All (ORCA). ORCA data provide travel behavior information that can be used to improve regional transportation system planning and decision making. The Data Science for Social Good (DSSG) program will be using two nine-week ORCA data sets, each consisting of over 20 million transit boarding records, to determine the changes in transit behavior that occurred when light rail stations were opened in the Seattle Capitol Hill and University District neighborhoods.
For example, we know that transfers from the Sounder commuter train to Link light rail more than doubled in spring 2016 when two new light rail stations opened. How many of these individuals are headed to the University District? Are they new transit riders? Or did they previously take buses to the University of Washington (UW)? If they took buses, did they take the limited direct bus service, or take the bus routes that operate more frequently to downtown, and then transfer to buses headed to the UW? We will also look at service characteristics before and after the light rail opens (e.g., are transfers to and from trains faster and more reliable than transfers to the UW bound buses?) to get a better understanding of why these behavior changes are occurring. The project goal is to not only describe the changes in travel behavior, but to develop scalable algorithms and procedures from our analysis that can be applied throughout the region. Can we develop reliable models that predict the different behaviors based on the different service levels of each type of trip? And if so, how different are those models from the ones currently used by Puget Sound Regional Council to predict transit use?
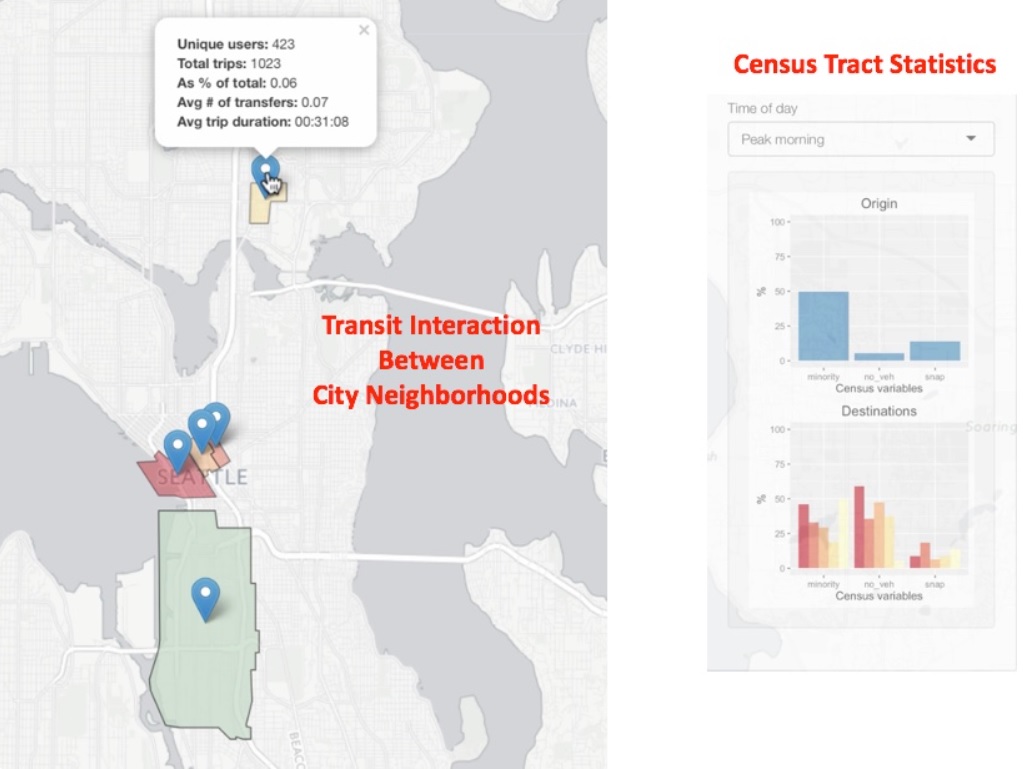
Project Outcomes: The ORCA DSSG group made several advancements. The first major advancement is an improved determination of origin/destination travel patterns from ORCA data. The second advancement was in improving estimation of total transit ridership, based on ORCA ridership.
The DSSG team developed a semi-supervised machine learning approach to improve the origin/destination matrices developed using ORCA fare card boarding data. The machine learning algorithm identifies which “transfers” marked bv ORCA actually represent transit boardings which have taken place after an activity has been performed, and which are direct transfers from one transit vehicle to another. The machine learning process identifies when two short trips are being treated by the ORCA financial system as one trip with a long duration “transfer” in the middle of that trip. This additional data processing step helps improve the accuracy of the ORCA data when describing where and when people are traveling via transit.
The second advancement entails a better understanding of how to estimate the number of cash paying customers using transit. ORCA payment records do not observe cash paying customers. The DSSG team explored multiple techniques for using ORCA data to estimate total ridership. The DSSG team explored several options. The most promising results from the summer involved the use of a Hidden Markov Model, which continues to undergo refinement.
View the Final Presentation and Final Poster.