Project Lead: Elaine Nsoesie, Institute for Health Metrics and Evaluation, Department of Global Health, UW
Data Scientist Leads: Valentina Staneva (primary) and Joe Hellerstein (secondary)
DSSG Fellows: Michael Munsell, Kiren Verma, Cynthia Vint & Kara Woo
Project Goals: The Centers for Disease Control and Prevention estimates that 48 million people experience foodborne illness, 128,000 are hospitalized and 3,000 die from foodborne illness in the United States each year. The estimated economic cost of foodborne illness is more than $15.5 billion annually. Early identification of unsafe food products would limit the occurrence of large foodborne disease outbreaks, thereby preventing illness and deaths, and limiting the health and economic impact on households, businesses and the food industry.
In this study, we aimed to investigate whether text mining of food product reviews can aid in the identification and ranking of food safety issues. Specifically, we focused on assessing whether text mining of the millions of consumer reviews posted online can be useful for early identification of unsafe food products that have the potential to cause foodborne disease outbreaks. The two aims of this project were: (1) mine and integrate a large corpus of data posted online to understand trends and features in unsafe food product reports, and (2) develop a machine-learning/informatics approach for early identification of unsafe food products. The data sources considered for this project include recalls of food products from the FDA, and USDA and online product reviews.
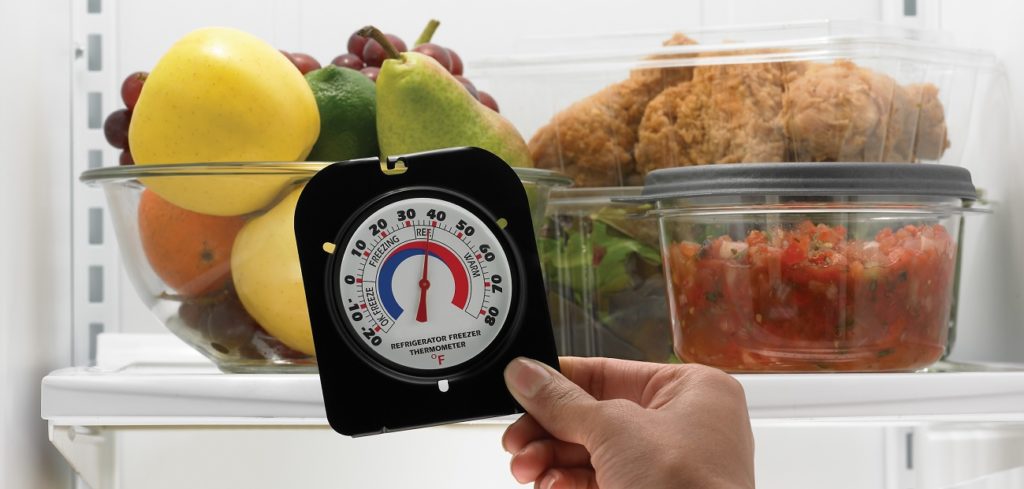
Project outcomes: We created an exploratory tool for viewing reviews of recalled products. We used Amazon reviews of Grocery and Gourmet Food products and enforcement reports from the Food and Drug Administration. The reviews in this tool provide some support for the idea that product reviews can be a fruitful data source for identifying unsafe foods.
There is still wide margin for improvement, and we need custom designed algorithms to extract the right features. However, initial exploration of the text showed that there exist features that indicate necessity for recall. It is a matter of selecting the right features that add weight to the most important aspects of the text.
We performed exploratory analysis of other aspects of the data in hopes of implementing into a better classification model. We investigated ways to implement the product categories as a feature in order to account for all of the product-specific noise. Also, we researched the corresponding FDA data and developed useful topics from the Reason for Recall text data. We have yet to determine if these are worthwhile features to include. Stay Tuned!
Stakeholders: Stakeholders include the general public, local and national public health entities, the Food and Drug Administration (FDA), and the United States Department of Agriculture (USDA).
Project webpage: https://uwescience.github.io/DSSG2016-UnsafeFoods/