By Emily F. Keller
Sept. 18, 2019
On Wednesday, Aug. 21, fellows from four interdisciplinary teams presented the results of their projects at the eScience Institute’s Data Science for Social Good (DSSG) program. The ten-week summer program, which began in 2015, brings together student fellows, stakeholders, and data and domain researchers to work on collaborative projects for societal benefit. Final presentations can be viewed here on our YouTube page. Project results are summarized below.
ADUniverse: evaluating the feasibility of (affordable) accessory dwelling units in Seattle
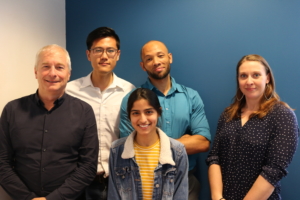
The City of Seattle partnered with the DSSG on this project to produce a feasibility tool for homeowners interested in creating an accessory dwelling unit (ADU) on their property for rental income, as space for visitors, or to house family. The development of the prototype tool is part of a broader effort by the City to encourage the creation of affordable ADUs across the city. Seattle Mayor Jenny Durkan mentioned the project in an executive order following the passage of legislation in July encouraging the development of ADUs, which include ‘attached’ units within a main house (AADUs), or ‘detached’ stand-alone units (DADUs).
The fellows were Yuanhao Niu, Adrian Mikelangelo Tullock, Emily A. Finchum-Mason, and Anagha Uppal. They worked with project leads Nick Welch, a senior planner at the Seattle Office of Planning and Community Development, and Rick Mohler, an associate professor in the UW Department of Architecture and a member of the Seattle Planning Commission, and data science lead Joseph Hellerstein, a senior data science fellow at the eScience Institute and an affiliate professor of computer science and engineering at UW.
The team utilized technical tools such as Python, SQL, and GIS to work with open-source data from the King County Assessor, OpenStreetMap and the City of Seattle’s GIS data, along with financial data from Zillow. The project’s Github page uses open-source code. The prototype tool addresses design, permitting, construction and financing issues, with a calculator to estimate construction costs, property loans, monthly payments and predicted changes in assessed property value. An interactive map shows eligibility data for constructing a DADU, aggregated by neighborhood, combined with population and income statistics. Users can enter their address to see the locations of ADUs in their neighborhood.
In addition to serving homeowners, the tool has research, education, and analytical applications. The team’s research showed the following initial results:
- An aggregate city analysis showing the number of AADUs and DADUs by neighborhood found some of the highest concentrations were in the areas of Greenwood and Green Lake.
- Only 2% of the 114,000 residential single-family lots in Seattle have an AADU, although all are eligible. Only 0.7% of the 107,000 lots eligible for a DADU have one. (Note: these totals contain overlapping counts, as some homes are eligible for both).
Learn more on the project website.
Developing an algorithmic equity toolkit with government, advocates, and community partners
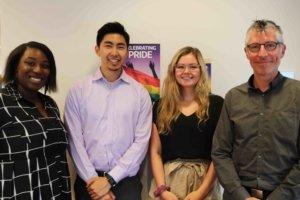
This project created a three-part toolkit to help civil rights organizations, members of the public and government officials gain a stronger understanding of the capabilities and social impacts of surveillance technologies and algorithmic decisions systems (ADSs). In particular, the toolkit is designed for use by civil rights activists to question and critique governments about their adoption and use of technologies through the public comment process. The fellows were Corinne Bintz, Vivian Guetler, Daniella Raz and Aaron Tam. The team’s leadership consisted of project lead Mike Katell and community engagement lead Meg Young, Ph.D. candidates at the UW Information School; data science lead Bernease Herman, a data science fellow and research staff member at the eScience Institute; and faculty advisor Peaks Krafft, a senior research fellow at the Oxford Internet Institute.
The toolkit was developed in partnership with the American Civil Liberties Union (ACLU) of Washington. The team engaged in a participatory design process, testing the tool for usefulness and clarity with civil rights organizations, and for accuracy of technical information with data scientists. The two primary stakeholder organizations were Densho, which works to preserve the history of the World War II incarceration of Japanese Americans, and the Council on American-Islamic Relations, which enhances understanding of Islam and promotes civil rights, justice, and empowerment for American Muslims. The team held several focus groups for additional feedback using the Diverse Voices method developed by the UW Tech Policy Lab. The group themes were: race and social justice, undocumented immigrant rights, and post-incarceration support leaders.
The toolkit has three parts:
- An interactive web demo helps organizations explore potential risks of surveillance and ADS technologies through a case study showing how inaccuracies and biases can occur in facial recognition technology. The demo uses an open-source dataset of celebrity photos and matching images selected by an algorithm through the software package OpenFace. Comparing several subjects, the demo shows that the software generates a higher rate of false positives for those who are black than those who are white.
- An ID guide enables users to identify specific technologies and understand their functionalities. The guide uses a flow chart to distinguish ADS from surveillance technologies based on easily identifiable features and clear definitions.
- A questionnaire provides a list of talking points for requesting specific information about technologies from public officials. Topics include transparency of documentation about how a tool was designed, data security measures, and the groups most likely to be affected by its use. Example cases are included.
I-405 high occupancy toll lanes: usage, benefits, and equity
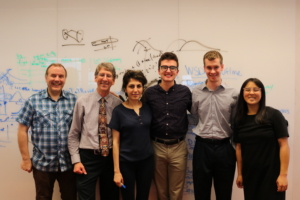
Through a partnership with the Washington State Department of Transportation (WSDOT), this project examined the impacts of congestion pricing on Interstate 405 with a focus on equity. Student fellows C.J. Robinson, Kiana Roshan Zamir, Shirley Leung, and Cory McCartan worked with project lead Mark Hallenbeck, director of the Washington State Transportation Center at UW, and data science lead Vaughn Iverson, a research scientist at the eScience Institute.
I-405, which runs from Bellevue to Lynnwood, has free general-purpose lanes, as well as high-occupancy toll (HOT) lanes that operate from 5, am to 7 pm, saving drivers about 30 minutes during peak periods. High occupancy vehicles can use the HOT lanes for free, while single-occupancy vehicles can access the lanes by paying between 75 cents and $10, depending on congestion levels. Tolling began in 2015.
To generate their analysis, the team worked with de-identified and aggregated tolling data provided by WSDOT that represented vehicle account registrations for all HOT lane trips in 2018, which totaled 16 million trips. To ensure account privacy, WSDOT de-identified the data by removing names and salting and hashing the account and license plate numbers, and reduced the resolution of the account locations by geocoding the addresses and assigning the resulting locations to census block groups. The team then used the aggregate data to estimate median income and population data for travelers, which they used to infer individual characteristics through a method called ecological regression. Then they modeled travel time savings and reliability in terms of dollar amounts, added these together, and subtracted the toll cost to calculate net benefits that are comparable across different income groups. Speed and volume data from loop detectors along I-405 helped shed light on time savings and reliability.
Results:
- HOT lane usage and average net benefit per household over all combined trips increases as income rises, though most HOT lane users are not high income (almost 80% have an estimated household income below $200,000).
- Low-income households travel more at peak periods when tolls are higher than during off-peak hours. As a result, they receive more net benefit per trip in terms of travel time and reliability savings than higher-income households.
- Frequent users have lower incomes than infrequent users. Those who use the HOT lanes daily have a median income of $81,000, compared to $101,000 for weekly users, and $114,000 for monthly users.
Natural language processing for online peer support
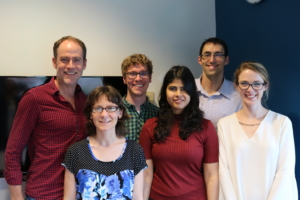
This project examined social media and online peer support for young adults who face mental health struggles. Fellows Kelly McMeekin, Shweta Chopra, and David Nathan Lang worked with data science lead Valentina Staneva, a senior data scientist at the eScience Institute. The project leads were Tim Althoff, an assistant professor in Computer Science & Engineering, and Dave Atkins, a research professor in Psychiatry and Behavioral Sciences, both at UW. The team began by citing statistics: Young adults from age 18-25 are more likely to face mental health struggles than any other age group, yet they are the least likely group to seek mental health care. Apps and websites targeting mental health have vastly increased in the last few years, but research about their impacts is limited.
This project studied one peer support platform with the research question: ‘What types of responses are the most helpful to individuals sharing their mental health struggles online?’ They analyzed a database of over 200 million rows of public conversations, user behavior and demographics, which they reduced to 2 million posts by removing social media content and focusing on peer support content for the year 2018. The team used a supervised learning model with a logistic regression classifier to label combinations of posts and comments as helpful if they contained one or more of these indicators: ‘likes’, ‘follows’, expressions of gratitude, or a change of mood, as selected from a list of categories. The indicators serve as proxies for helpfulness in lieu of more direct measures that are unavailable from an online platform, such as professionally annotated data, surveys or mental health assessments. The platform has 500,000 users, the majority of whom are female, with a median age of nineteen.
The team utilized a natural language processing (NLP) technique called “topic models” to categorize content themes. For those seeking help, the most common topics ranked by frequency were despair, emotions, social relationships, anxiety, family/ daily life, social media, appearance, anger/rage, and mental health. The comment topics in response to posts, ranked in order of frequency, were relationships/appearances, broad encouragement, social circle, reflection/summary, stay strong, personal outreach, emotions, family/daily life, self-care, distraction, and social media/frustration. To compare online peer support with traditional counseling techniques, the team tagged their dataset with a trained classifier. More details are available on the project website.
Results:
- The user characteristics that were associated with higher rates of helpfulness were being female, younger, and having large networks.
- Three out of four behaviors from traditional counseling that appeared in the online platform – affirmations, reflections, and summaries – were positively correlated with helpfulness proxies, while the open questions technique was negatively correlated with helpfulness proxies
- Users whose posts were of a more critical or serious nature were overall less likely to indicate that responses to their posts were helpful.
- Based on words and language used in posts, using affirming and encouraging phrases, and making oneself available for others to talk to were perceived as helpful, while short acronyms like lol or jk, or negative language such as the word don’t were perceived as less helpful.
- Comments that were longer, contained emojis, and spoke to the person seeking help in the second person (versus talking about one’s self in the first person) were perceived as more helpful.