The eScience’s annual Data Science 2024 Incubator program kicked off last week, which enables new research discoveries by bringing together data scientists and domain scientists to work on focused, intensive, collaborative projects. Our team of data scientists provide expertise in state-of-the-art technology and methods in large-scale data manipulation and analytics, cloud and cluster computing, statistics and machine learning, and visualization to help researchers extract knowledge from large, complex, and noisy datasets. In the ten years since the Incubator program launched, eScience has collaborated on over 65 total projects from a hugely diverse range of UW departments and units. See the archive of past Incubator projects here.
This year, we selected six projects to further explore data science as it applies to the areas of glacial lake flooding, cancer risk genetic variants, the United Nations, shallow convection patterns, Alzheimer’s Disease, and wildfire impact on park visitation.
“Characterizing glacial lake outburst flood hazard at regional scale using fused InSAR-speckle tracking surface displacement time series”
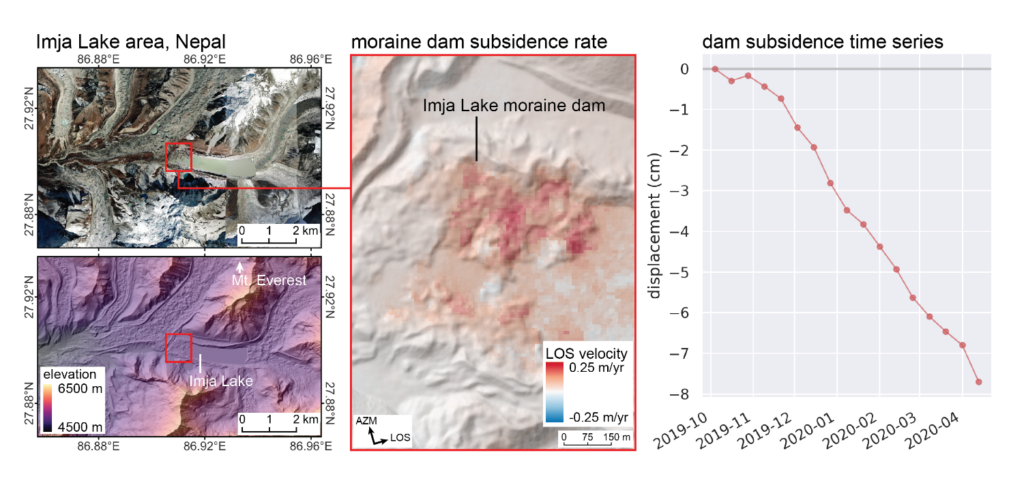
Project Lead: George Brencher, UW Civil & Environmental Engineering
Data Science Lead: Scott Henderson
Glacial lakes are distributed in alpine terrain worldwide and are frequently dammed by unstable glacial moraines. These moraine dams can fail, causing lakes to rapidly drain and flood downstream valleys. Glacial lake outburst floods (GLOFs) are a significant hazard for high-elevation infrastructure and communities—on October 4, 2023, a GLOF in Sikkim, India, destroyed the Teesta III hydroelectric dam, washed away 15 bridges, affected hundreds of villages, stranded 3,000 tourists, and left at least 74 dead with many more missing. This flood was triggered when a landslide on a glacial moraine catastrophically failed and fell into the South Lhonak Lake, causing it to breach its banks. The landslide had been moving downslope at rates of up to 10 meters per year since at least 2016, but was not identified prior to its collapse, despite multiple flood hazard and risk analyses for the site.
Using satellite synthetic aperture radar remote sensing, we have developed a workflow allowing us to quantify surface changes that can contribute to GLOF likelihood, including landslide movement and moraine dam subsidence. Our approach fuses interferometric synthetic aperture radar (InSAR) and SAR speckle tracking data to accurately capture deformation as fast as hundreds of meters per year and as slow as <1 cm per year. For this incubator project, we hope to improve and scale our workflow to measure surface displacement with high spatial and temporal resolution for the areas surrounding all of the large glacial lakes in Nepal for the length of the Sentinel-1 archive (~2016-present). The resulting multi-year displacement time series will allow us to detect and track intra- and inter-annual changes of dynamic landslide, permafrost, and glacial features. We will also precisely quantify rates of moraine dam subsidence, significantly improving our understanding of GLOF hazard for hundreds of dangerous lakes and providing a critical missing input to existing risk analysis frameworks. In addition, we expect that 1) our scaled approach will be easily transferred to other regions, allowing us to create robust regional displacement time series anywhere on Earth, and 2) our almost decade-long, high spatial and temporal resolution displacement time series will be of broad scientific interest to glaciologists, geomorphologists, engineers, and hydrologists working in mountainous environments.
“Investigating germline genetic influence on somatic immune traits in non-cancerous tissue”
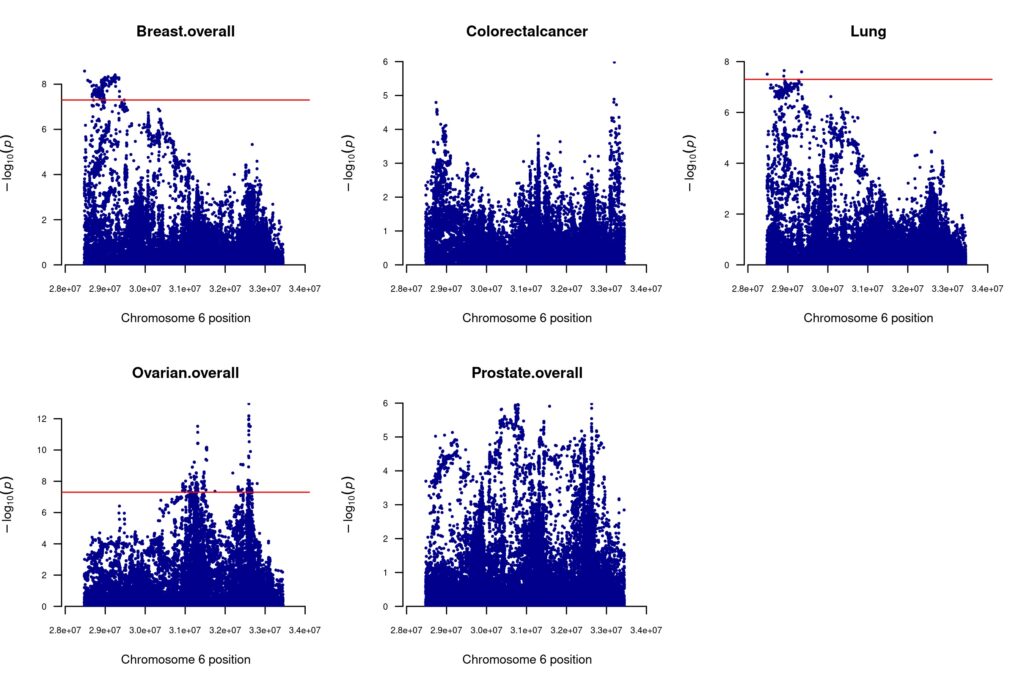
Project Lead: Tabitha Harrison, UW Epidemiology
Faculty Advisor: Sara Lindström, Epidemiology
Data Science Lead: Vaughn Iverson
Cancer is a significant health burden in the US, causing substantial morbidity and mortality. This research project builds on past studies demonstrating that inherited genetic variants can influence how our immune system affects cancer risk and survival. While prior work has linked aspects of the immune system to tumors, this project focuses on understanding how our inherited genetic makeup affects immune function in healthy tissues. Using publicly available data from dbGaP and GTEx, we will examine how inherited immune-related genetic markers (in the HLA region) relate to immune function in non-cancerous tissues in the lung, breast, prostate, and colon. We will then investigate if genetic marker variations that affect immune traits in healthy tissues are associated with developing common solid cancers.
“What do the leaders say? Analysis of the United Nations General Debate Corpus”
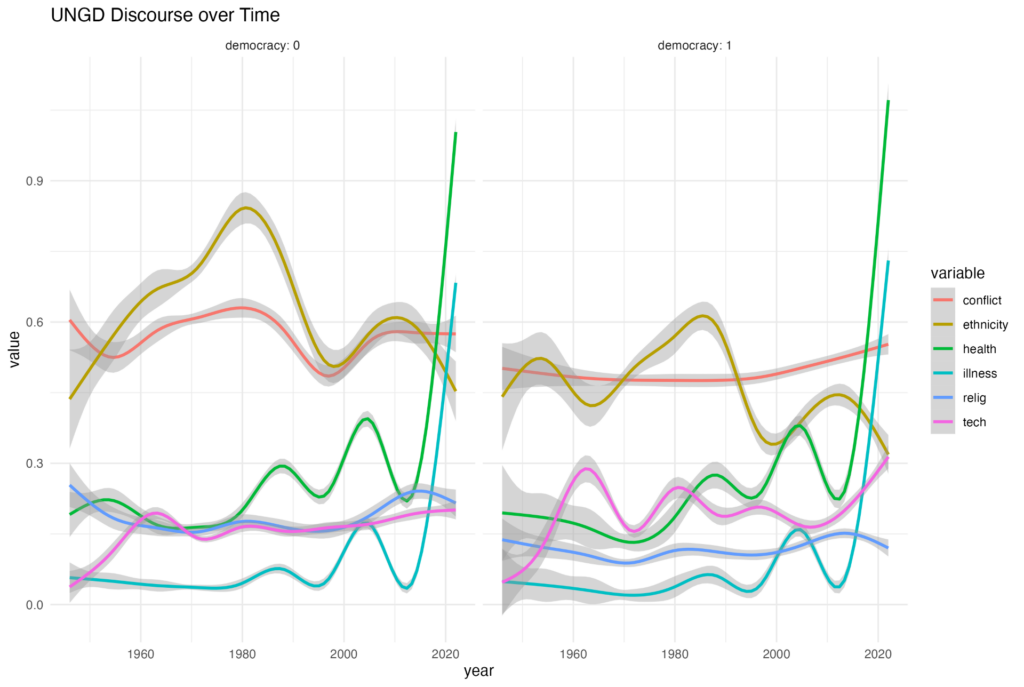
Project Lead: Jihyeon Bae, Ph.D. Candidate, Political Science
Data Science Lead: Valentina Staneva
Every year, more than 200 state representatives open the United Nations General Assembly meeting with statements during the General Debate (UNGD). The UNGD speech floor provides a rare opportunity for all states to equally have their voices heard by a wide range of audiences. In addition, UNGD statements are crucial factors that set the agenda for the remaining sessions and incentivize states to condense their viewpoints concisely. This project analyzes a large text corpus containing 10,568 English transcripts of speeches delivered by state representatives of UN member states at the United Nations General Debate from 1946 until 2022.
In the first stage, we pose a testable hypothesis: “How do democracies and autocracies frame the principle of sovereignty differently?” Sovereignty is the most fundamental legal principle in the realm of global governance, developed to guarantee legally equal status among states and respect authority over territories. However, authoritarian states have invoked the sovereignty principle, framing it as a free pass to enact any policies domestically. We aim to determine if there is any systematic difference in rhetorical usage between the two types of regimes, using text analysis models. In the next stage, we analyze not only what the leaders say, but how they speak by employing computational linguistics models. Our goal is to unpack the preferences of authoritarian state leaders by mapping UNGD data to psychological markers. This project is expected to contribute to the timely discussion on the growing political clout of authoritarian regimes.
“Illuminating the role of cold-pools in structuring shallow convection”
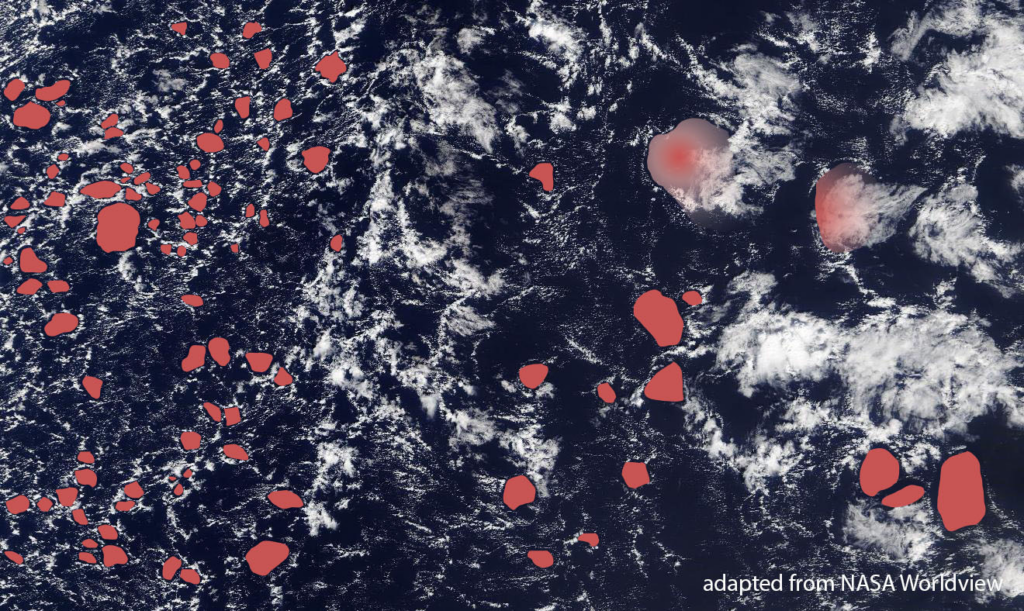
Project Lead: Hauke Schulz, Cooperative Institute for Climate, Ocean, and Ecosystem Studies (CICOES)
Data Science Lead: Bernease Herman
Shallow convection, like the stratocumulus decks off the Washington coast, is responsible for a large portion of the uncertainty in climate projections, thus a better understanding of their processes is crucial. Advances in computational resources allow for ever increasing resolutions of climate simulations, yet the resolutions remain too coarse to simulate these clouds and their underlying processes explicitly. Parameterizations – simple algorithms or empiric relationships that estimate the unresolved processes based on the resolved processes – need to be refined with the increase in resolution as they no longer hold true. To develop these new parameterizations, the formation processes of these shallow clouds need to be understood at finer and finer detail. A detail that is currently left out in these parameterizations is the fact that these clouds can occur in a variety of spatial patterns. To simulate these clouds and their cooling effect correctly in the current and future climate, these patterns are crucial to represent correctly.
In order to develop a better parameterization of these clouds in our climate models, we need to improve our understanding on how these different patterns of cloudiness form. Previous studies suggest that precipitation drastically influences these patterns, in particular through the generation of so-called cold pools. These cold pools (marked in red in the satellite image) that are areas of cold air and form due to the evaporation of precipitation are able to redistribute clouds by suppressing them within the cold pool and generating new convection at their edges. The identification of these cold pools in satellite observations will provide valuable information to better understand the formation of different cloud patterns and ultimately lead to an improved parameterization of shallow convection.
Here we utilize several data sources to generate ground-truth cold-pool labels and train a neural network that is capable to identify individual cold pools in satellite imagery.
“Polygenic and Contextual Determinants of Alzheimer’s Disease and Related Dementias”
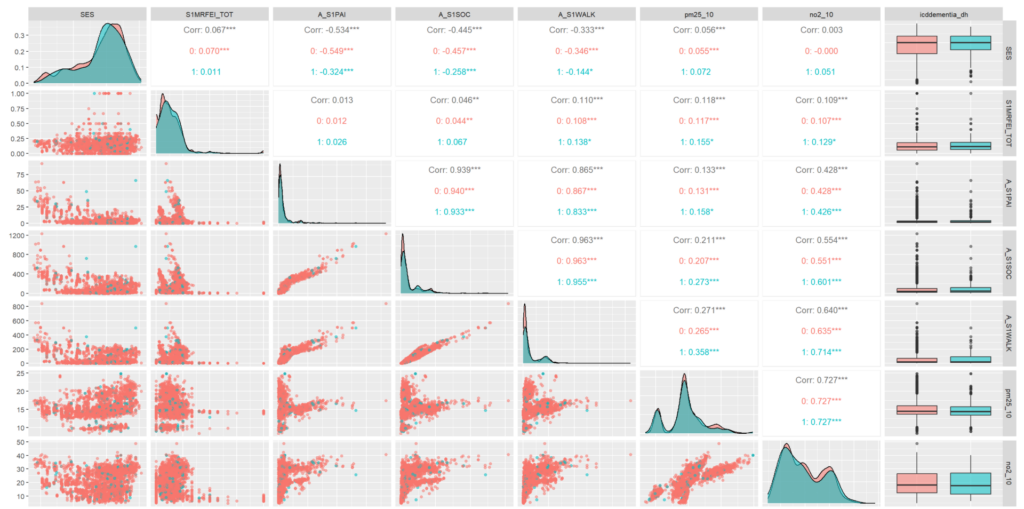
Project Lead: Diane Xue, Institute for Public Health Genetics
Data Science Lead: Bryna Hazelton
One in three people over the age of 65 dies with dementia. The most common cause of dementia is Alzheimer’s disease (AD), a progressive neurodegenerative disorder influenced by genetic and environmental factors. Dozens of genetic loci have been linked to AD and related dementias, and there is growing evidence that social, built, and physical environmental factors are associated with dementia outcomes. Yet, few studies have investigated the effects of social, built, and physical environmental factors after controlling for polygenic risk for AD.
The goal of this project is to model multi-level macro- and meso- environmental factors including ambient pollutants, socioeconomic status, density of physical activity facilities and social engagement destinations. alongside polygenic scores that summarize individual-level genetic risk for AD in order to determine what social and environmental factors remain significantly associated with dementia risk and/or cognitive decline after controlling for PRS. Additionally, we want to investigate whether effects of social and environmental factors differ for high- and low- genetic risk groups. Social, built, and physical environmental variables that are associated with healthy controls who are at high genetic risk can be further investigated as population-level solutions for promoting AD resilience. Furthermore, early prediction of AD is key to prevention. The results of the proposal will prepare us to integrate genetic and non-genetic factors for risk prediction, moving us close to precision treatments.
“Assessing Influences of Wildfires on Park Visitations Patterns Using Gravity”
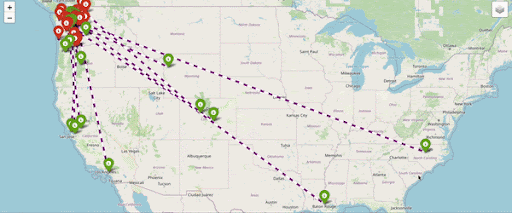
Project Lead: Nino Migineishvili, UW Computer Science and Engineering
Data Science Lead: Spencer Wood
Wildfires have been growing in size, duration, and destructivity, resulting in more decisive calls to improve forest health and protect communities. Wildfire fuel treatments – which involve reducing or removing vegetation from fire-prone areas – are one strategy for reducing wildfire risk. Where to conduct fuel treatments implementation is primarily based on biophysical risk factors. Yet wildfires also disrupt recreational utilization of public lands, which in turn affects societal well-being and the numerous physical and mental health benefits of recreating in nature. Given this, the aim of this project is to develop approaches for estimating recreation on public lands and quantify how recreationalists respond to wildfires and wildfire treatments on the landscape. The approach uses gravity models with trips to public lands sourced from AllTrails.