The eScience’s annual Data Science Incubator program kicked off last week, which enables new research discoveries by bringing together data scientists and domain scientists to work on focused, intensive, collaborative projects. Our team of data scientists provide expertise in state-of-the-art technology and methods in large-scale data manipulation and analytics, cloud and cluster computing, statistics and machine learning, and visualization to help researchers extract knowledge from large, complex, and noisy datasets. In the nine years since the Incubator program launched, eScience has collaborated on over 60 total projects from a hugely diverse range of UW departments and units. See the archive of past Incubator projects here.
This year, we selected six projects to further explore data science as it applies to the areas of snowmelt, acoustic sensing, mass-spectrometry, wetland communities, marine heatwaves, and social science.
“Leveraging large satellite archives to understand the timing and distribution of global snowmelt”
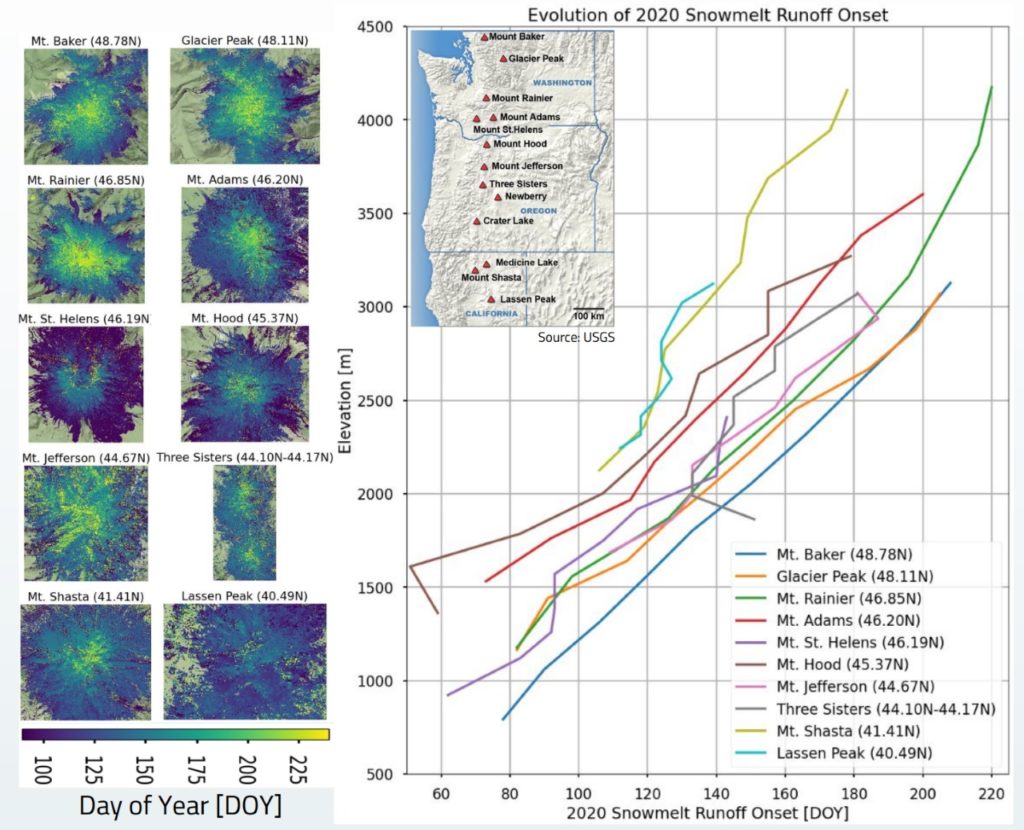
Project Lead: Eric Gagliano, Terrain Analysis and Cryosphere Observation Lab, UW Civil & Environmental Engineering
Faculty Advisor: David Shean, UW Civil & Environmental Engineering
Data Science Lead: Scott Henderson
Seasonal snow plays an essential role in the Earth system and more than 1/6 of the world’s population relies on runoff from seasonal snow and glaciers for agricultural and domestic water supply. Snowmelt timing has important implications for downstream water resource applications, flood risk management, and ecosystem maintenance. Snowmelt timing is often used as a common indicator of regional climate change: for the Western U.S., snowmelt timing is projected to advance earlier in the year by up to one month by 2050. The snowmelt runoff onset date dictates both the beginning of increased water availability and the rate of spring flow. High-resolution maps of snowmelt runoff onset date would enable more accurate water resource forecasting and regional climate analysis.
Synthetic Aperture Radar (SAR) microwave instruments on satellites offer high spatial and temporal resolution measurements and provide the ability to delineate between different snowmelt phases. I developed and released an open source toolbox to explore cloud-hosted publicly available Sentinel-1 C-band SAR data and produce snowmelt runoff estimates. This method analyzes the dielectric properties of water in snowpack over time to determine when the snowpack is saturated and runoff onset begins. So far, I have primarily focused on analyzing time series for smaller sites such as the Cascade Range Stratovolcanoes. I’ve been able to produce snowmelt runoff onset maps and analyze controls (such as topography and weather) on runoff timing at high resolution, which is currently poorly understood in mountainous areas. However, with additional guidance and computational resources, I hope to scale up my methods to create snowmelt timing products on a regional to global scale. These products will be valuable for water resource managers to better understand when and where snowpack releases water into critical reservoirs, as well as climate scientists looking to analyze regional trends in snowmelt timing as an indicator of regional climate change. The objective of this incubator project is to scale up the processing of these snowmelt runoff onset maps beyond the current watershed and mountain scale. My primary goal is to process the full Sentinel-1 catalog (2014-present) to prepare seasonal snowmelt runoff onset maps for the Western U.S., with a final stretch goal of processing the entire globe.
“The Prototype of a Cloud Store for Distributed Acoustic Sensing Data”
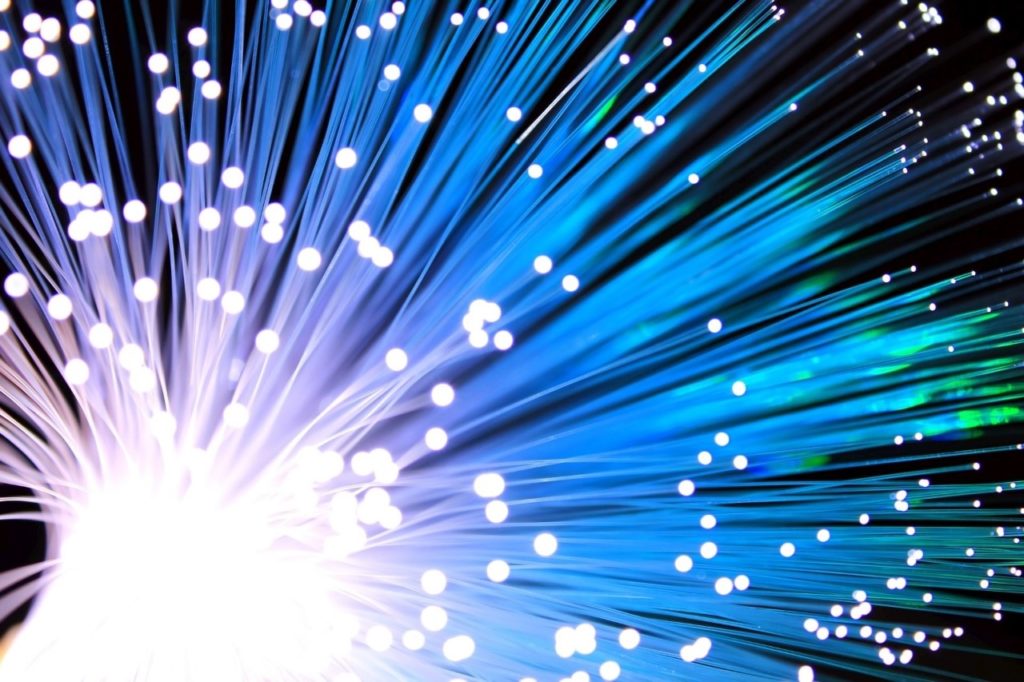
Project Lead: Yiyu Ni, Earth and Space Sciences, UW College of the Environment
Data Science Leads: Naomi Alterman and Rob Fatland
Distributed Acoustic Sensing (DAS) is the recording of strain measurements distributed along an optic fiber cable using photonic sensing. DAS can record signals from cars, trains, ships, planes, earthquakes, volcanic tremors, avalanches, footsteps, and whales. The technology is a revolution for seismology research, with great potential for frontier applications such as wildlife monitoring and the built environment. The data comes in at a high rate (100-10kHz) and multi-channels (100s-10,000s) continuously. The Photonic Sensing Facility (PSF) at UW already has 100sTBs of DAS data from 3 PSF-related experiments. At the same time, the PSF is planning several short- and long-term experiments that will bring the data archive to 1PB by the end of 2023. This large amount of data necessitates the exploration of the data format and metadata structure for fast data queries and processing. This incubator project aims to pilot the first cloud object storage to host DAS data. Our team will deploy a local cloud storage service on our local servers to emulate a cloud platform for DAS research using cloud-optimized data formats. The outcome of the project will be a pilot experiment promoted for authoritative seismic networks and archives.
“Constructing a robust metric of peak quality for untargeted mass-spectrometry”
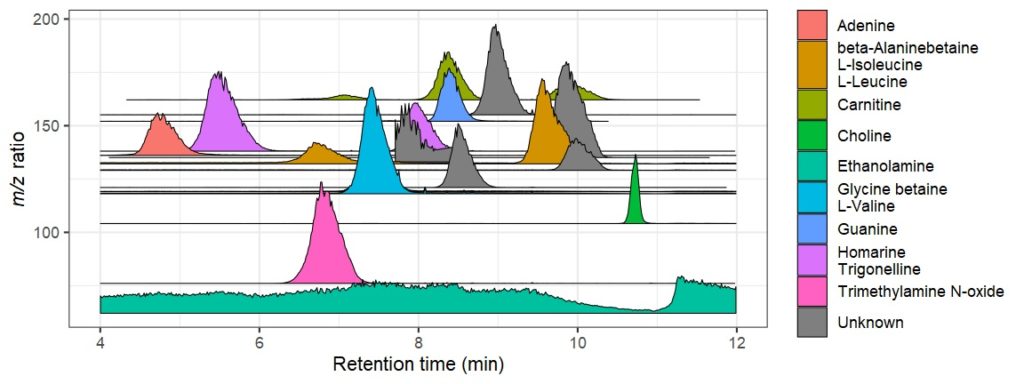
Project Lead: Will Kumler, UW Oceanography
Faculty Advisor: Anitra Ingalls, UW Professor of Chemical Oceanography
Data Science Lead: Bryna Hazelton
Mass spectrometry is a cutting-edge analysis field used to identify the molecular composition of samples taken from medical laboratories, the depths of the ocean, and even outer space. In the Ingalls Lab at UW, we use it to characterize the molecular composition of seawater and its inhabitants, a task complicated by the complex biogeochemistry of the oceans. The nascent nature of modern mass spectrometry also introduces many challenges, one of which is distinguishing biological/chemical signal from noise produced during the measurement process.
My goal in this incubator is to calibrate existing detection algorithms to a probabilistic likelihood that the signal corresponds to a real molecular feature. This will involve estimating the relative strength of various metrics used for detecting molecules, using machine learning methods to construct the probabilistic estimate, and ideally constructing packages that interface with existing software to facilitate widespread adoption.
“Wetland Communities in the US”
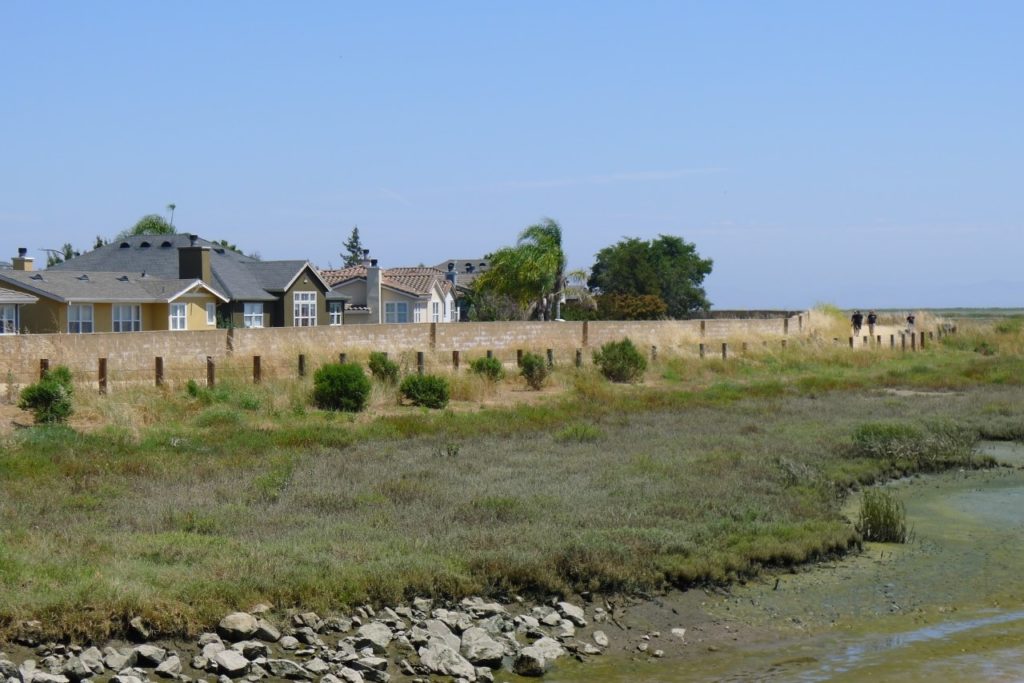
Project Lead: Celina Balderas Guzman, UW Assistant Professor of Landscape Architecture
Data Science Lead: Spencer Wood
Sea level rise threatens human communities on the coast. Conserving coastal wetlands is increasingly seen as an adaptation response that can protect human communities by absorbing storm surge. How many people currently benefit from the protective benefits of coastal wetlands? Who are they? This research seeks to quantify and profile “wetland communities” in coastal states using data science techniques. Global institutions, national and local governments, non-profits, practitioners and scientists are currently promoting wetland conservation as an adaptation response. This increased interest means investment dollars and planning resources are going into wetland conservation. The motivation behind this work is to provide useful information for researchers, policymakers, and communities interested in wetland restoration or protection and prioritizing projects.
“Characterizing the spatio-temporal evolution of marine heatwaves”
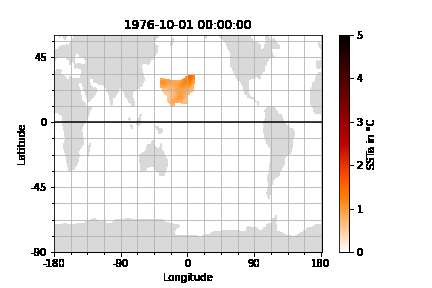
Project Lead: Cassia Cai, UW Oceanography
Faculty Advisor: LuAnn Thompson, UW Oceanography
Data Science Lead: Valentina Staneva
Marine heatwaves (MHW) are discrete events characterized by periods of anomalously high sea surface temperatures (SSTa). MHWs can have significant ecological and socio-economic impacts, such as leading to algal blooms, habitat degradation, and loss in commercially valuable fisheries. In the last two decades, a number of high-profile MHWs, such as the Great Barrier Reef 2022, Mediterranean Sea 2003 and 2006, Tasman Sea 2015, Northwest Atlantic 2012, and Northeast Pacific 2013-2015, have occurred. In a warming world, MHWs are expected to be more frequent and more intense. In this project, we develop different metrics to understand the diversity of MHWs and a workflow that picks features to classify and find patterns in the spatio-temporal evolution of MHWs. This can help fill multiple knowledge gaps, such as our understanding of key MHW characteristics like distribution, variability, and trends, and the physical mechanisms that cause MHWs in different parts of the ocean.
“Investigating Structure of Social Science Research Datasets for Better ML Evaluation”
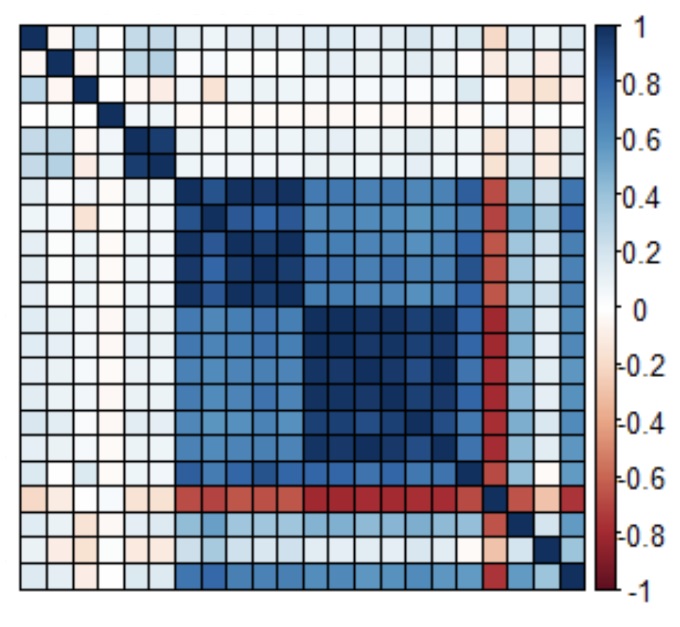
Project Lead: Bernease Herman, eScience Data Scientist
Specialized machine learning architectures, such as deep learning, typically rely on inductive biases and other data-specific correlational structure information to produce more effective models. Similarly, the design and evaluation of differentially private synthesizers depends heavily on the correlational structure of the datasets most commonly used in the field. We wish to investigate differences in the correlational structure of popular machine learning benchmark datasets with those of other disciplines who utilize machine learning, starting with social science data. We will both investigate the structure by repurposing existing descriptive dataset metrics in addition to exploring new graph-based metrics that generalize well across many data types.

By: Louisa Gaylord
Louisa is the Communications Specialist at the eScience Institute, where she writes about data science and its applications across a wide range of fields. She also manages weekly direct email campaigns, social media, and website updates, creating websites for eScience groups and other technical writing projects.