August 6, 2019
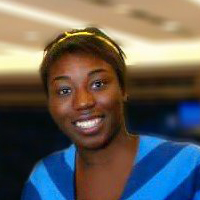
Bernease Herman, eScience data scientist, has been awarded a $25,000 Mozilla Research Grant (2019H1) for her project titled “Toward generalizable methods for measuring bias in crowdsourced speech datasets and validation processes.”
The project aims to measure dataset bias in the Mozilla Common Voice crowdsourced speech collection process and dataset. Given the goals of the Common Voice project, measures of diversity and bias are essential indicators to understand, measure, and report. This is already reported on the validated dataset across number of languages, number of unique speakers, regional accents, age, and gender.
The primary contributions of this project are:
1. Characterizing utterances that were not validated to understand dataset validation bias and inform crowdsourcing procedures for speech
2. Exploration of generalizable speech diversity measures that reduce the need for categorical measures of diversity and generalize across languagesHerman wishes to research an understudied perspective on exploring and mitigating dataset bias by honing in on data validation processes. It is often assumed that most dataset bias is caused by pulling data from sources that are not representative of the intended population. There is literature on crowdsourcing for subjective labels [1], but the tasks are often explicitly subjective such as emotional affect. Written text processing systems that have undocumented preprocessing steps to remove misspelled words are one example of a validation process.
Common Voice has a validation process wherein two (of possibly three) annotators must validate that utterance matches the written sentence. The proposed guidelines use a number of examples to encourage validators to accept variation so long as each word of the sentence is pronounced and correct [2], making the task seemingly objective. But the overall validation step has the potential to introduce lots of bias in the dataset. Common Voice’s crowdsourced speech process explicitly outlines the intended validation step and makes unvalidated utterances available publicly, making for an ideal initial point of study and potential benchmark dataset for the machine learning and speech communities.