UW DSSG 2024 Projects
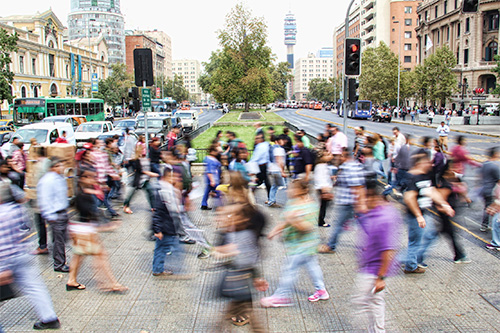
Crowd-Flow Project
Measuring Fairness and Equity in Crowd-Flow Generation Models
Generative crowd-flow (CF) models are machine learning models that are capable of producing mobility flow resembling population movement in a city. Such models traditionally come from physics models that were fundamentally based on association of distance, population and propensity of travels. Recently, new crowd flow generation models based on neural networks have emerged. These models can incorporate additional information from the city (such as amenities and reviews) and have been shown to outperform older models. However, to date there is little evidence as to whether any of these new models can create synthetic data that results in equitable flow prediction for all parts of society or whether they exacerbate social biases by widening the representation gap. These concerns are pressing given that government agencies often consider crowd-flow models in planning for public safety, traffic management, and other critical areas of operation.
Fairness is increasingly recognized as a critical component of machine learning (ML) systems, but little attention has been given to the applications of city planning and urban related research that directly relies on spatial temporal data. We argue that understanding fairness in practice relies on observing a model’s behavior in the context that is intended to be used.
In this DSSG project, we will develop definitions of group fairness of GenAI-CF models. Such definitions could help us to measure the equity of CF synthetic datasets. By incorporating a more equitable representation of under-served groups’ travel demands, our project aims to ensure that future transportation policies, infrastructure investments, and service improvements are informed by a comprehensive understanding of all community needs.

Homelessness Project
Understanding Unsheltered Homelessness in King County: UW 2023 Seattle Area Homeless Count
The US Department of Housing and Urban Development released the 2023 Annual Homeless Assessment Report (AHAR) on December 15, 2023. The report estimates that 653,100 people in the U.S. experienced homelessness in 2023, a 12% increase from 2022. This estimate comes from the Point-in-Time (PIT) count conducted on a single night in January over communities across the US. The PIT, which is mandated by HUD every two years, is composed of two key elements: (1) the emergency shelter report from administrative records and (2) the unsheltered PIT count typically performed on a single night in January through volunteers walking around the community and tabulating how many people they see. This so-called “visual census” of unsheltered people experiencing homelessness has a number of issues, from methodology (people are undercounted for a number of reasons) to ethics (people don’t get a voice in how they are counted). In other words, there is much room for improvement in understanding our unhoused neighbors.
A team at UW led by Dr. Zack Almquist has been working with the King County Regional Homelessness Authority since 2022 to improve the unsheltered PIT methodology and accompanying demographic and needs assessment surveys. In 2022, the University of Washington and KCRHA implemented a novel, network-based method for counting the unsheltered people experiencing homelessness known as Respondent-Driven Sampling. That was followed by a larger pilot study conducted in 2023, resulting in a dataset containing rich network and demographic information from 1,100+ sheltered and unsheltered people. This year’s DSSG project will finalize the 2023 data set and conduct analyses to better understand the needs of people experiencing homelessness, especially those living in vehicles. The team will produce policy reports for KCRHA and create an outward-facing website to host the findings and describe the method for other communities to use.
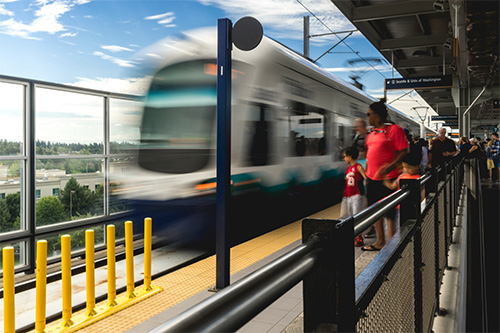
Transit Equity Project
Investigating Transit Equity Through ORCA Fare Card Analysis
Transit service is a public good, and quality service for all transit users, especially transit-dependent populations, is essential for improving access to opportunities. Transit agencies typically lack knowledge about transit usage patterns. Understanding current gaps and inequities in service can help transit agencies improve service, encourage increased use, and reduce environmental impacts. Transit fare card data describes actual transit use. Its analysis allows assessment of the equity of the existing transit system, can describe gaps in services being provided, illustrate specific locations where effective improvements need to be made, and identify changes in route structure that would better serve riders.
Fare card data is a form of trace data which can be used to identify individuals and their movements through time and space. This project will access and analyze fare card data from the ORCA system—the regional electronic payment system used by transit agencies throughout the Puget Sound region in Washington State. ORCA is a massive and sensitive dataset that allows for analysis of large numbers of people over multiple months. ORCA has specific classes of fare cards, allowing for identification and analysis of trip characteristics for specific types of priority transit users such as users with subsidized passes due to low-incomes or disabilities (e.g., frequency and number of trips and transfers, wait time for transfers, type routes used). By examining actual transit use of anonymized riders against geographic and demographic characteristics, we can identify where transit system improvements need to be made (e.g., new routes to better serve important movements, changed route structures to lower transfer times, transit stop improvements such as shelters where many people transfer) to improve the quality of services being provided, with emphasis on demographic populations most dependent on transit.

Water Reuse Project
Constructing a Drivers-Based Framework for Assessing Water Reuse
Communities around the United States are thinking of alternative water systems to address local water challenges. One example of this is water reuse, which is defined by the Environmental Protection Agency (EPA) as “the practice of reclaiming water from a variety of sources, treating it, and reusing it for beneficial purposes.” The current social problem is that communities only see water reuse as an opportunity for areas that are experiencing water scarcity, rather than realizing it’s full potential to address a wide range of water challenges, like reducing combined sewer overflows, minimizing the nutrients that are discharged to the environment, and lowering flood risk.
Our project aims to address this social problem by developing a framework for quantifying a community’s potential for water reuse based on various motivators—or drivers—to identify whether water reuse could be a local solution that merits further investigation. Combining that data into an informative index and presenting the results in a clear and digestible format is critical for supporting local decision-making. Using publicly available data across the US, our project looks at the correlation between drivers (both presence and intensity) and characterizes the benefits communities might find by exploring water reuse. Outcomes from this work will be synthesizing these relationships into an interactive storymap for effective, real-world use of the research by local communities, engineers, and decision-makers.