Project Lead: Bernease Herman, eScience Data Scientist
Specialized machine learning architectures, such as deep learning, typically rely on inductive biases and other data-specific correlational structure information to produce more effective models. Similarly, the design and evaluation of differentially private synthesizers depends heavily on the correlational structure of the datasets most commonly used in the field. We wish to investigate differences in the correlational structure of popular machine learning benchmark datasets with those of other disciplines who utilize machine learning, starting with social science data. We will both investigate the structure by repurposing existing descriptive dataset metrics in addition to exploring new graph-based metrics that generalize well across many data types.
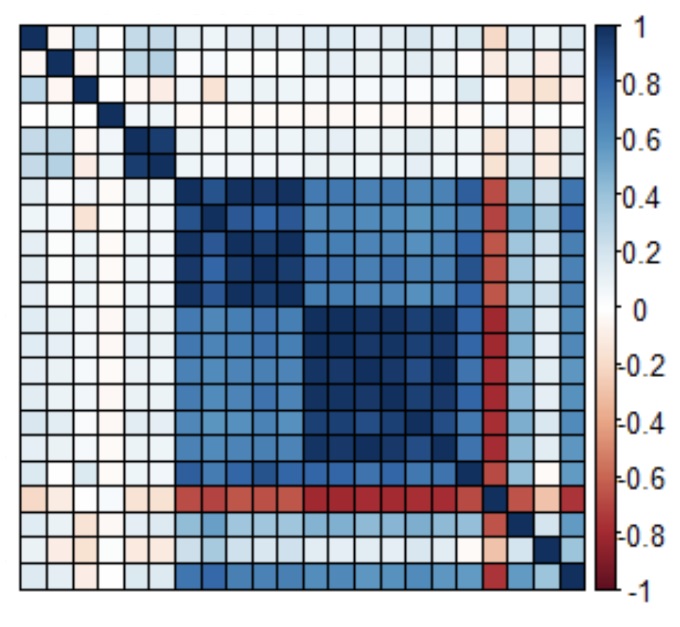