Project Lead: Jennifer Hsiao, Department of Biology
eScience Liaison: Bryna Hazelton
Over the next three decades rising population and changing dietary preferences are expected to increase food demand by 25–75%. At the same time climate is also changing — with potentially drastic impacts on food production. Breeding for new crop characteristics and adjusting management practices have the potential to mitigate yield loss due to a changing climate. However, identifying optimum plant traits and management options for different growing regions through traditional breeding practices and agronomic field trials is time and resource intensive. Mechanistic crop simulation models can serve as powerful tools to help synthesize cropping information, set breeding targets, and develop adaptation strategies to sustain food production.
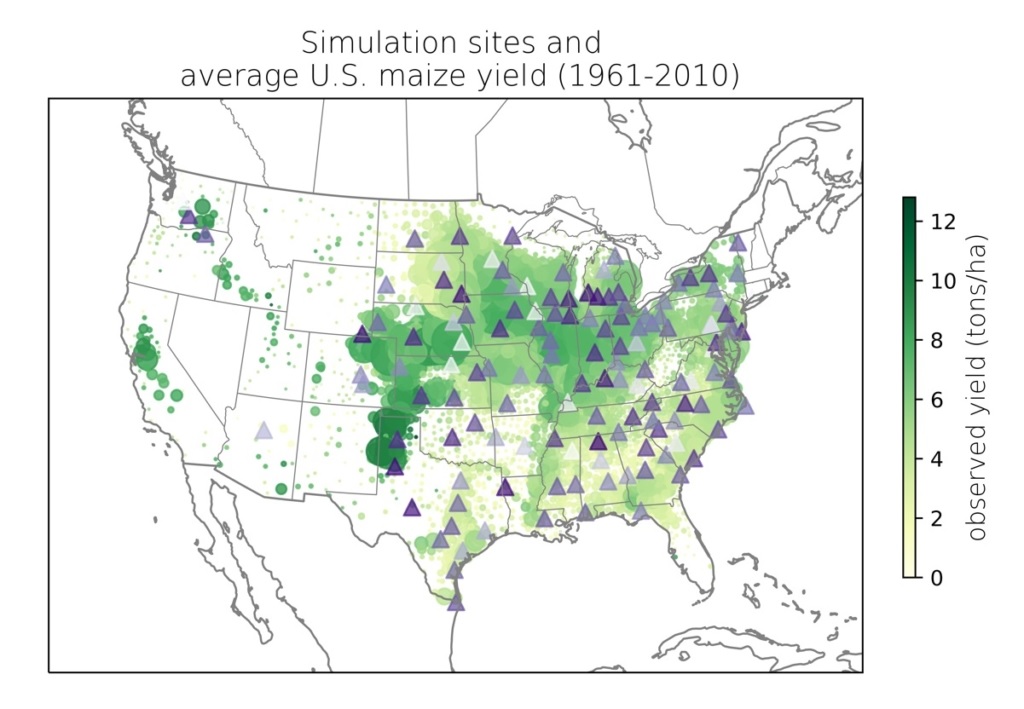
In this project, we use a mechanistic crop simulation model to explore how different crop traits and management options affect maize growth and yield, with the hope to identify ideal trait and management combinations that maximize yield and minimize risk for different agro-climate regions in the US.
We identified various sites across the US maize growing region that had several years of hourly growing season climate information available as environmental drivers for the model (Fig. 1). We then set up an ensemble simulation for each simulated site-year that perturbed physiological, phenological, and morphological aspects of the modeled maize plant and recorded the final yield and various growth processes throughout the growing season. We identified key plant traits that were important for high yield, and observed how the importance of traits differed amongst growing regions.
This quarter-long collaboration yielded four main deliverables:
- We overhauled and modularized much of the original project code to set up a well-documented and tested data-model pipeline, which allowed us to easily pre-process input data required for model runs and build reproducible workflows for different simulation experiments;
- We developed a SQL-relational database that stores all ensemble model outputs, which allowed for easy query, analysis, and visualization of large amount of model output that spans time, space, and model parameter perturbations;
- We incorporated a sensitivity analysis framework to identify key model parameters (plant traits) that contribute to high yield either through calculating the partial correlation coefficient or by performing the Fourier amplitude sensitivity analysis;
- Finally, we identified plant trait combinations that led to high yield and low yield volatility, and showed plant trait combinations that performed best across different maize growing regions within the US. We found that plant traits linked to crop phenology and development had the greatest impact on contributing to high yield under current climate conditions. We also identified some regional differenced in best performing cultivars between cooler northern regions vs. warmer southern regions.
We are currently setting up new simulations that further perturb identified key plant traits together with management practices such as planting date and planting density to identify ideal plant trait and management combination, and to investigate how these ideal combinations could shift in different growing regions under a changing climate. We hope this work will shed light on region-specific adaptation strategies for US maize facing a changing climate.
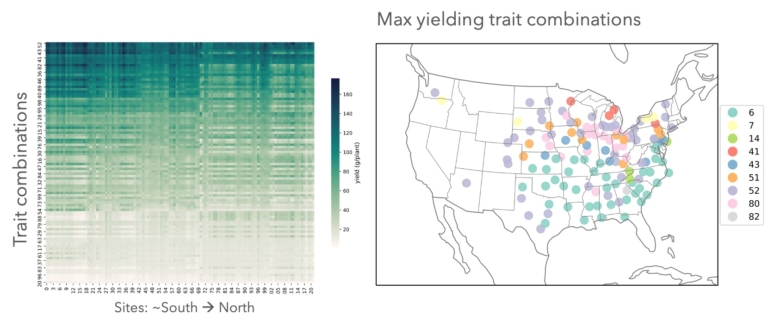