Project Leads: Christina Bandaragoda, senior research scientist, Watershed Dynamics Research Group, Civil & Environmental Engineering; Purshottam Shivraj, Data Science Master’s Student; Nicoleta Cristea, research associate, Mountain Hydrology Lab, Civil and Environmental Engineering
eScience Liaisons: Amanda Tan and Rob Fatland
Sparcity of temporal data in high elevations is a hurdle for every water resources researcher investigating hydrologic processes in watersheds with greater than 1000 m rise in elevation. Temperature is interpolated to high elevations from low elevation measurements as a lapse rate (C/km), are correlated to precipitation (rain or snow) and short and long wave radiation. Across the continental United States, an annual average temperature lapse rate of 6.5 C/km is commonly used; in the Pacific Northwest, where clear-sky assumptions do not hold, an annual average temperature lapse rate of 4.8 C/km has been reported (Minder et al, 2012). Although annual average constant lapse rates are representative for the long term average hydrologic response, the sensitivity and importance of sub-daily, sub-watershed distributed mountain microclimatology and atmospheric mechanisms that control temperature lapse rates for capturing atmospheric river, rain on snow, and snowmelt driven flood events is not well understood. Interpolated and gridded shorter time scale lapse rates (e. g., daily) based on low elevation weather observations (COOP stations) are generated at continental scales with no local control on lapse rate assumptions. We need to understand the tradeoff between capturing long term watershed behavior (annual or monthly average) and short term events dependent on high elevation atmospheric interactions (with limited observations).
To address this problem we plan to develop a process to update gridded hydrometerology climate forcings at a watershed scale using local datasets, interpolating across elevation ranges based on theoretical and empirical relationships (e.g. Unsworth and Monteith, 1975). We will use data collected beginning with 2015 by the researchers from the UW Watershed Dynamics Research Group and the Nooksack Indian Tribe. The temperature observation field campaign is across a North Fork Nooksack transect of Mt. Baker (600-1800 elevation range). These results show that 3hr time series of lapse rates vary +/- 5 C/km from an 4.8 C/km annual average based on precipitation (or clouds indicated by relative humidity. Our proposed methods are to use Dakota software (Adams et al, 2015), and a DiffeRential Evolutionary Adaptive multi-objective optimization algorithm (DREAM; Vrugt, 2016) to calibrate a Landlab (Hobley et al, 2017) hydrometeorology component that uses low elevation observations (following Livneh et al, 2015) and physics-based lapse rates to match high elevation atmospheric model (WRF; Henn et al., 2017; Currier, 2016; Currier et al., 2017) monthly averages, from a cloud computing environment (e.g. HydroShare). The feasibility of our proposed process is supported by the fact that our team and projects (PREEVENTS, Landlab, HydroShare) are published authors in each field of expertise; during this incubator we will combine existing utilities to address this problem.
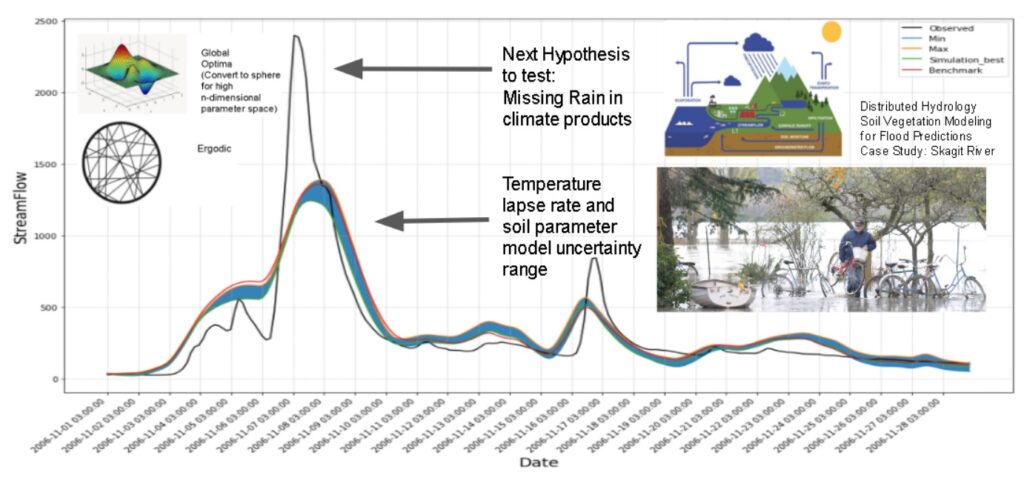