Project lead: Benjamin Brooks, UW Institute for Health Metrics and Evaluation
Advisor: Abie Flaxman, UW Institute for Health Metrics and Evaluation
eScience Liaison: Andrew Whitaker, UW eScience Institute
There has been considerable attention given to the potential for search engine and social media data to provide real time information regarding public health threats; this idea is well known in the context of influenza. Public opinion concerning vaccination is of interest since the publication of a study in 1999 (now discredited) linking the measles, mumps, and rubella (MMR) vaccine to autism; in its wake, parental fear of vaccination has risen, vaccination rates have decreased, and occurrence of outbreaks of vaccine-preventable diseases have increased. Relative to other applications of social media data in public health, the study of anti-vaccination sentiments is particularly appropriate given that individuals are often opinionated on the topic and might be expected to share such opinions publicly.
We are interested in using Twitter data as a means of monitoring general anti-vaccination sentiment. In particular, we hypothesize that opinions shared on Twitter regarding vaccination provide insights into where geographic clusters of anti-vaccination sentiment exist, and, consequently, where children are not immunized and outbreaks might be expected. A study published in 2011 used a series of keywords to identify and collect Twitter data related to vaccination over a six month period after the H1N1 (“swine flu”) vaccine became available to the public. The researchers developed a classifier by compiling a training dataset where students tagged tweets as containing positive, negative, or neutral sentiment toward the vaccine for about 10% of their data; this classifier was then used to categorize the rest of the tweets into one of the three bins.
While this study showed that users with anti-vaccination opinions tended to cluster within the social network, it only used a crude measure to validate whether those opinion manifested themselves in measurable outcomes of public health concern. They used geographic information associated with individual Twitter accounts to compare the average “sentiment ratings” of different regions of the US to H1N1 vaccination rates and found a reasonably strong positive correlation (i.e., more positive sentiment, higher vaccination coverage). Our goal is to extend this work by examining whether these clusters can be linked to particular geographic areas at the state or, preferably, sub-state level and whether those areas have experienced outbreaks of vaccine-preventable disease since the original link between autism and the MMR vaccine was published.
We tested this hypothesis by combining vaccination-related Twitter data with data published through the National Notifiable Disease Surveillance System, which provides weekly case counts of newly diagnosed cases of key infectious diseases (including those that are preventable through vaccine) for each state [18]. In the process of working towards this goal, we tested several different sentiment classification methods, collected a new body of vaccination-related Twitter data from 2014, and examined whether the average sentiment expressed on Twitter in 2009 during the H1N1 pandemic was similar to the average sentiment in the same geographic areas in 2014.
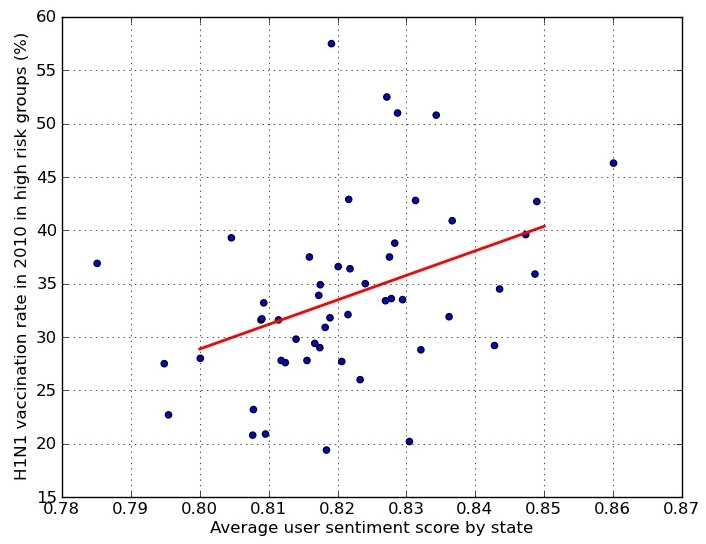