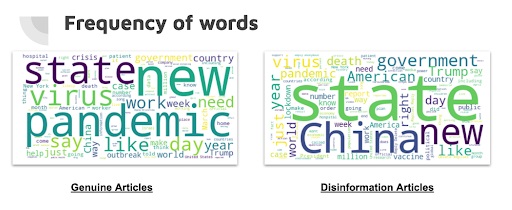
While informative news articles about COVID-19 play a key role in fighting the pandemic, a range of erroneous blogs and articles with fake virus cures, political propaganda and conspiracy theories are also circulating online.
A new open source tool being developed as a project in the eScience Institute’s Data Science for Social Good (DSSG) summer program will help identify disinformation articles, as part of a larger effort to disincentivize its content producers and reduce its spread.
The tool is being designed in partnership with the nonprofit organization Global Disinformation Index (GDI), whose mission is to defund disinformation sources that are inadvertently supported by “ad tech” (advertising technology) companies that post advertisements to websites automatically without a systematic process for reviewing the website content. This results in companies and organizations unintentionally placing their ads on disinforming websites, which in turn profit from the ad placements. The tool will contribute to GDI’s efforts to warn ad tech companies about high-risk websites that have a large quantity of disinformation articles.
The DSSG program brings together students, stakeholders, data scientists and domain researchers to work on project teams for a 10-week period. Identifying Coronavirus Disinformation Risk on News Websites is one of two projects hosted by the program this year. The project team consists of eight people: four Student Fellows from universities around the country, two eScience data scientists who provide technical guidance, as well as Lead Data Scientist Maggie Engler and Senior Researcher Lucas Wright as the two project leads from GDI.
One of the team’s first steps was to define the problem area. “Defining disinformation was something that our team discussed at length, because it is a concept that can be subject to different interpretations,” said Maggie Engler. For example, the term ‘disinformation’ often refers to articles created with intent to deceive, which would be difficult to detect from content alone. Instead, the Student Fellows are building a model to identify what GDI calls an ‘adversarial narrative.’ “Rather than simply classifying true or false information about the pandemic, they’re trying to detect narratives that commonly leverage false information to sow conflict and promote division,” explained Lucas Wright.
To develop the model, the DSSG team is looking for a set of features that distinguish disinformation articles from genuine news, based on tens of thousands of blogs and articles that GDI has scraped from the web and labeled through automatic and manual processes. They are conducting a literature review to assist with methodology decisions and using machine learning techniques to train the model, which will classify articles based on their probability of containing disinformation about the coronavirus.
Interdisciplinary backgrounds are an asset in this complex project, which draws on a variety of skills, such as sentiment analysis, natural language processing, and bias mitigation. Student Fellow Kseniya Husak, a master’s student in public policy and information science at the University of Michigan, discussed the benefits of working with a diverse group. “Given my background in public policy, my first instinct is to evaluate as many potential outcomes as possible. That means asking a lot of ‘what if?’ questions and evaluating alternatives. Working with an interdisciplinary team helped narrow down my approach to this problem and ground it in what is technically possible given the time constraint my team is working with,” she said.
To ensure that projects have their intended impact in the real world, the DSSG program engages project stakeholders early and throughout the program. The team met with two lead members of GDI to learn more about how the data they are working with was collected, the financial relationship between advertising networks and online content providers, and the environment in which their model is designed to be integrated. They will also meet with Jevin West, director of the Center for an Informed Public at UW, which combats misinformation through research, education, policy and public engagement.
Fellow George Hope Chidziwisano, a doctoral candidate in media and information at Michigan State University, described the influence of stakeholder engagement on his work. “At first, I thought the model we are developing will work independently to classify news articles. However – through stakeholder meetings – I have come to realize that models are not 100% accurate, thus human input will still be needed to reduce the risk of false positive results from the model,” he said.
Project Challenges and Processes
Generating a predictive model has raised many ethical considerations, beginning with the detailed decisions involved in pre-processing the data and their impact on the final outputs. Discussion topics have included how to treat website hosts and authors fairly when labeling content as disinformation, and how to design the model dynamically to be adapted as adversarial narratives evolve over time.
Fellow Richa Gupta, a master’s student in Quantitative Methods in the Social Sciences at Columbia University, said the program’s multi-layered approach, including stakeholder engagements, a literature review and discussions within the team highlighted the complexities of the project. “Starting from the abstract nature of the problem, and data collection challenges, to impact analysis of the outcomes of the work we will produce. Through this fellowship I have realized the importance of defining the problem before starting to solve it,” she said.
The team has had to carefully consider data cleaning processes often used to standardize data for machine learning – such as removing special characters, repetitive and non-informative words, duplicate articles with different URLs, and randomly capitalized text – as these might be features of disinformation content that could be useful in their analysis. To determine a set of disinformation features, they are looking beyond key words or phrases and considering potential indicators such as length of article text, semantics, spelling accuracy, domain name patterns and embedded hyperlinks.
Noah Benson, a Senior Data Scientist at the eScience Institute, described the unique challenge of identifying disinformation articles that, unlike weather or traffic data, are often designed to evade detection. “Simultaneously, the ethical ramifications of misidentifying valid information as disinformation are steep, so as a team we have had to reevaluate a lot of assumptions about what would and wouldn’t differentiate genuine articles from malicious ones. The deceptive nature of the problem has made having a diverse team and a broad set of outside perspectives especially valuable,” he said.
Vaughn Iverson, a Senior Research Scientist at the eScience Institute, discussed the team’s strategy for treating information sources fairly to prevent negative impacts that could result from an unsubstantiated label of disinformation. “The threshold for classifying disinformation needs to be very conservative, that is, heavily biased away from generating false positives. We recognize there is a strong asymmetry in the potential impacts here, so while a false negative classification means that some bad information on the internet lives to see another day, a false positive could potentially trigger a serious negative financial impact on an innocent party,” he said. In addition, work is progressively being conducted privately to prevent the public identification of content sources with unverified classifications, and plans are underway for a dispute resolution process and human oversight over automatic classifications.
Fellow Maya Luetke, a doctoral candidate in epidemiology at Indiana University, Bloomington, said her main takeaway from the project so far is the power of a “thorough, thoughtful, and iterative analysis of the subject matter, problem, and implications of our technology. The code will be written and the models will be trained and tested, but it really seems like this careful and comprehensive assessment of the complexity of a problem is what will make this project successful.”
The final DSSG 2020 presentations will take place on Wednesday, August 19th via Zoom from 1:00 to 2:30 p.m. The event is open to the public, please follow this link for more information and to RSVP.