Project Lead: Kwong-Yu Wong, UW Department of Economics
eScience Liaison: Jose Hernandez
Rationing is usually necessitated whenever some external constraints causing quantity of goods provided in lack of what is required (e.g. essential supplies in wartime, surgery needed, meals during peak hours etc.). In Economics literature, rationing is commonly regarded as welfare reducing because it easily induces wasteful competition such as wasting time by standing in line. However, whether rationing only induces wasteful competition is still an open question.
This project studies the beneficial competition under rationing in food delivery industry and helps quantify the welfare improvement resulting from the competition. In food delivery industry, rationing happens daily in peak hours. Such rationing induces customers to compete in calling in earlier for food delivery and hence restaurants (and delivery company) receive information earlier to have a larger flexibility in meeting the demand on-time. This will be one clear example of beneficial competition induced by rationing.
To quantify the overall welfare impact of such beneficial competition, we need to build a counterfactual where the beneficial competition is removed, in order to compare with the reality with such competition. One major technical challenge is to predict which delivery person will a delivery order be assigned to when the call-in time needs to be altered. Since customers compete in call-in time, artificially altering the call-in time removes the competition effect in counterfactual.
Once call-in time of an order is adjusted in counterfactual, how this order will then be assigned is unknown as this does not happen in reality. While we can naively guess the assignment by simply assigning the order to the closest delivery person, the assignment should in fact be much more complicated in considering factors such as their orders on-hand and the corresponding finishing time. This project aims at predicting the assignment with the help of statistical machine learning tool. With the assignment properly predicted, we can then simulate how the delivery will be completed in the counterfactual scenario and hence measure the outcomes (e.g. delay time in all delivery orders) to compare with the reality. The beneficial competition effect is then quantified by the difference in two scenarios.
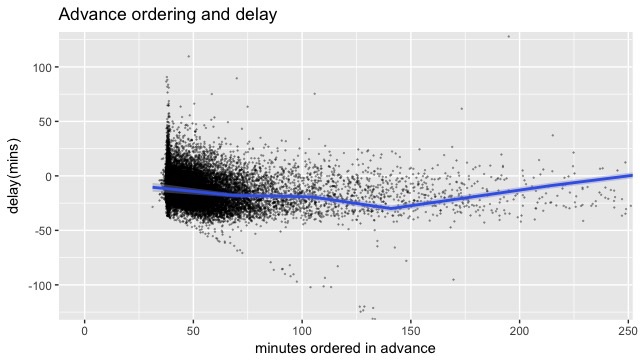
Project Results
Although the data on hand is rich, some key features for meaningful analysis are missing. Data only has addresses for customers and restaurants in Chinese. Latitude-longitude and travel distance are missing. Without such information, regression analysis on delay time is counter-intuitive. For example, the regression would suggest higher delivery fee induces a larger delay for delivery. To complicate things further, Google map in China is less accurate than the leading map in China, Baidu map. Typical API request through Google is not ideal. During incubator program, we’ve built a handy function to request Baidu API for geocoding information. Even though there is daily limit for Baidu API, the function helps to split the task across weeks to obtain the information. Enabled by these additional features, we can have better control variables for delay time analysis. Delivery fee increase, after controlling for distance and traffic complications, reduces delay time as one would expect.
Another concern for analysis is about the relationship between order time and eventual delay. While straight-forward regression would give us quick grasp on how data look like, one might be concerned if the delay effect roots in treated group (e.g. customers who place order 20mins earlier) and control group (e.g. customers who place order later) are totally different by nature. We supplemented the analysis with multivariate matching and propensity score matching. The former ensure the similarity between treated and control by choosing a corresponding counterpart in control group for each observation in treated group. Instead of choosing 1-1 counterpart, the latter estimated propensity score for weighting all observations in control group so as to make a group comparable to treated group. Both methods confirm the delay effect is significant in a comparable treated-control pair.
Going forward, we shall consider cost-benefit analysis so as to discuss how early should information be sent out to improve welfare. Instrumental variable would be needed for causality discussion. Since ordering time is endogenously optimized, its associated coefficient does not directly inform what would happen had ordering time been changed. Rainfall can serve such a role to disentangle cost side and benefit side because it affects only the delivery but not the ordering. With that in place, this project would be able to inform how early should the information be communicated to optimize on rationing.
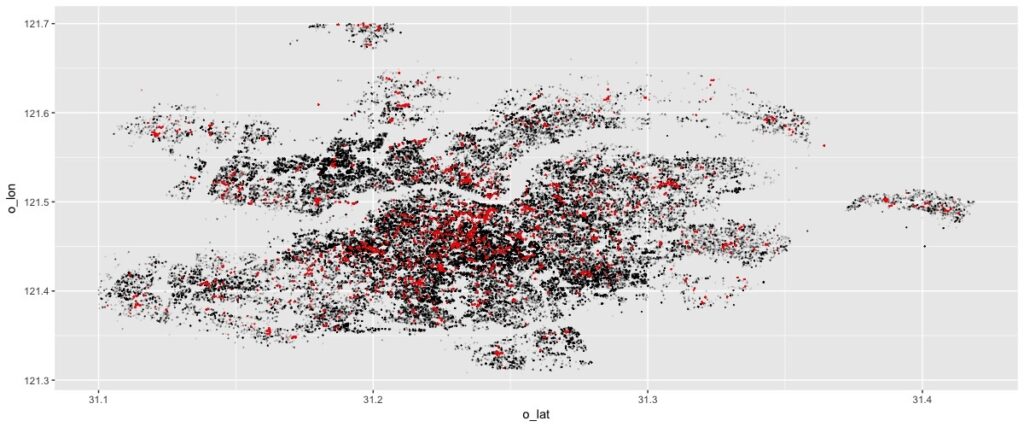