Project Lead: Wu-Jung Lee, Applied Physics Laboratory
eScience Liaisons: Bernease Herman and Valentina Staneva
The temporal and spatial occurrence of predator-prey interactions, and the associated biomass change across the food chain, are of central importance in the marine ecosystem. Compared to net-based sampling methods, sonar systems (echosounders) offer promising potentials for quantifying such interactions, by delivering synoptic observation of the whole water column at each ensonification (echogram; Fig. 1). The Ocean Observatories Initiative (OOI) recently deployed numerous such systems, with an ambitious goal of cross-trophic observation at a significantly longer time scale than previously possible. However, there are imminent challenges, since the traditional subjective and non-adaptive echo analysis methods are not effective for analyzing the continuous ocean observatories sonar data flow with limited biological ground truth information (e.g., species composition) at the majority of locations.
To overcome these challenges, we will develop a suite of data-driven machine learning and inverse methods for objective segmentation and interpretation of OOI sonar echo time series. This is a crucial step toward delivering foundational biological information to understand the marine ecosystems under the changing climate – a highlight of the OOI program. We will use multi-dimensional echo features, including the mean echo strengths, distribution of echo fluctuation, and their joint variation across frequency as data descriptors. Since these features are strong functions of the size and identity of marine organism aggregations, by learning patterns based on echo features, we parse the incoming sonar data stream into biologically meaningful groups (e.g., fish-zooplankton foraging assemblage) useful for ecological research. The analysis framework will be structured such that the data parsing rules are updated adaptively based on the temporal evolution of echoes in the data stream.
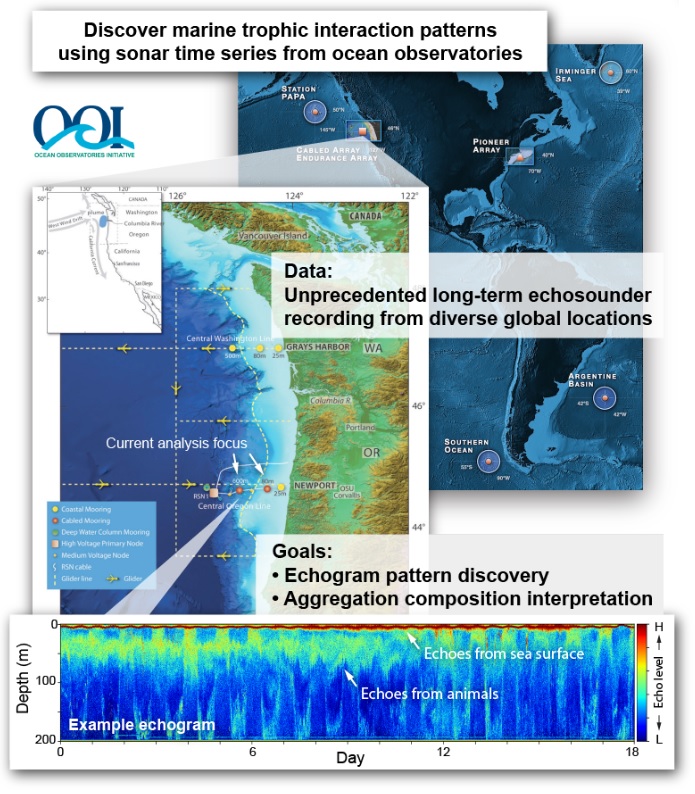