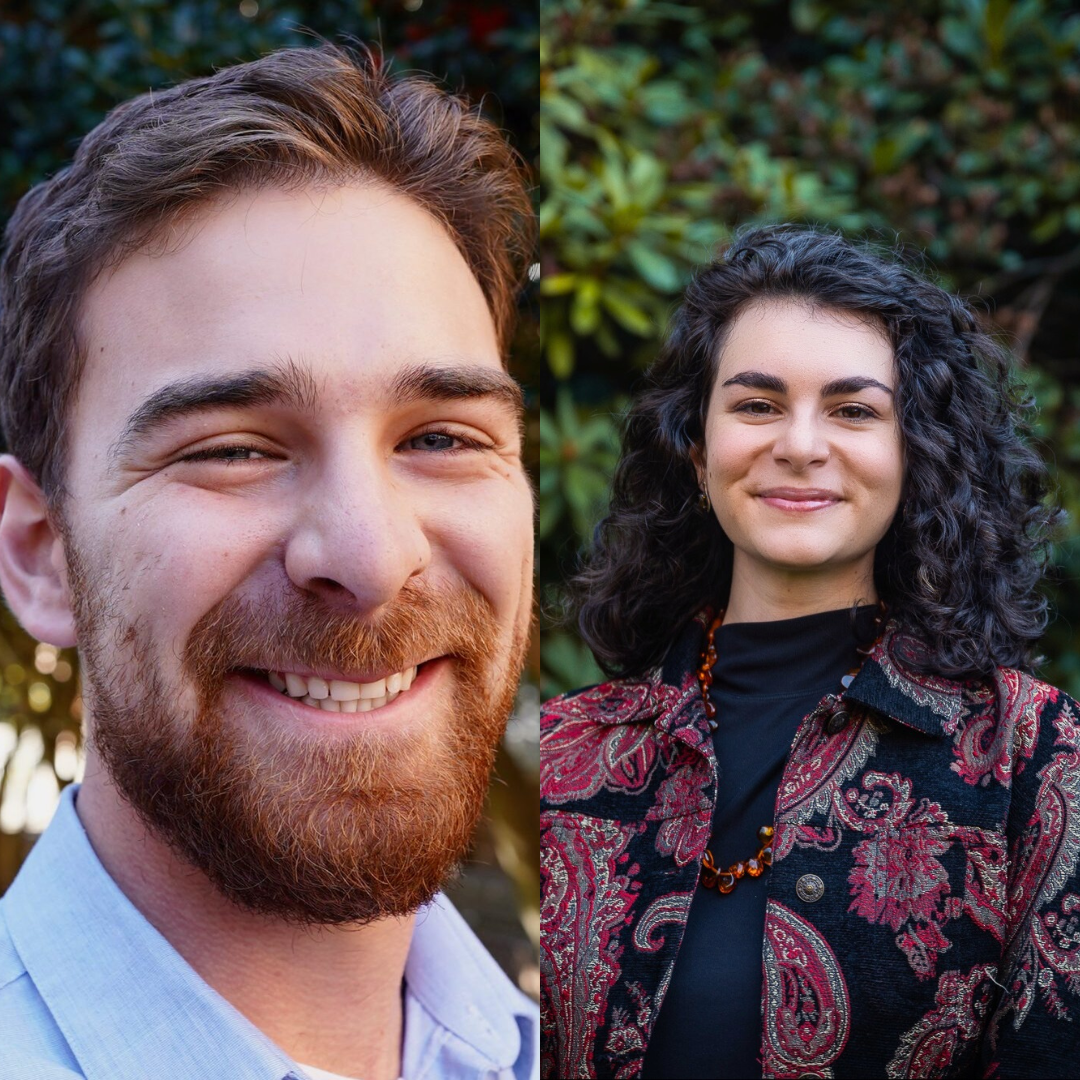
Please join us for a UW Data Science Seminar featuring UW Aquatic and Fisheries PhD student Ben Makhlouf and UW Environmental and Forest Sciences Masters student Masha Vernik on Wednesday, May 7th from 4:30 to 5:20 p.m. PT. This seminar will feature two different research projects supported by the eScience Data Science and AI Accelerator.
The seminar will be held in Electrical and Computer Engineering Building 125 – Campus Map.
“Developing machine learning models alongside multi-source biological data to conserve Alaska Salmon”
Abstract: Pacific salmon are vital to Alaskan communities, serving as a key cultural, subsistence, and economic resource. However, Chinook salmon populations in Western Alaska have declined sharply in recent years. While many hypotheses suggest marine mortality as a key factor, identifying the specific origins of fish caught in the open ocean has been a challenge, limiting the ability of researchers to explore hypothesis which may provide valuable insight into the causal mechanisms of recent declines and potential adaptation strategies to mitigate it. Isotopic markers in otoliths (ear stones) of pacific salmon offer a promising for estimating the location of origin for migratory fishes. These structures grow sequentially over the lifetime of the individual, continually recording a sequential record of experienced isotopic values into a timeseries of experienced isotope values. By isolating a discrete value from this timeseries which corresponds to a period of interest (e.g. juvenile rearing), researchers can relate this ratio to those modeled across the landscape to provide a precise estimate of provenance. While this method works well at relatively small spatial scales (within watershed), redundancy in isotope ratios at large spatial scales often fail to estimate precise locations using a single discrete value.
What has been relatively unexplored is whether the full timeseries of otolith data from the lifespan of an individual can overcome this limitation to estimate the location of origin. Because of the complex isotopic makeup of dendritic river systems, the full timeseries may constrain patterns which indicate watershed specific migration pathways. Further, the otolith shape itself may contain patterns which, when combined, aid in identifying the river-of-origin.
Here, we apply machine learning models to otolith timeseries data along with shape analysis using fourier transformations to explore the potential of building a model to distinguish river of origin in ocean-caught salmon. Preliminary analysis suggests otolith timeseries data, when deployed in Machine learning models such as Random Forest, may be able to correctly classify >75% of individuals. In addition, otolith shape analysis shows promise to further improve on this classification accuracy, particularly when both approaches are combined. If successful, a working model which can be deployed in Alaskan fisheries to test valuable hypotheses amid an ongoing crisis. In addition, this project highlights the potential of deploying machine learning and data science techniques alongside ecological data and integrating multiple data sources and modeling approaches.
Biography: Ben Makhlouf is a graduate student in the school of Aquatic and Fisheries sciences researching the spatial ecology of Pacific Salmon in Western Alaska. Namely, Ben is interested in developing and deploying new tools to address pressing conservation and research needs in the region by combining ecological datasets alongside emerging data science tools. Before graduate school, he worked for several years helping to run a field camp in southwest Alaska to help monitor salmon ecosystems.
“Bayesian Modeling to Understand Climate Adaptation Among Organic Farmers in the U.S.”
Abstract: As seasons become less predictable and extreme weather events become more frequent and intense, farmers learn to adapt. However, context-specific constraints may differentiate the extent to which different farmers facing the same environmental exposures are able to adapt. Specifically, results from a qualitative study with organic farmers in western Washington revealed that farmers with insecure land tenure are unlikely to make infrastructure investments necessary to adapt to changing conditions. This talk features preliminary results from a Bayesian approach to modeling the effect of land access on perceived adaptive capacity to climate change among organic farmers in the United States. The model is based on survey data collected by the Organic Seed Alliance and Organic Farming Research Foundation integrated with meteorological data measuring drought, temperature extremes, and climatic variability. The talk addresses how to incorporate results from qualitative studies into quantitative modeling approaches through variable and prior selection, offering insight into mixed-method studies.
Biography: Masha Vernik is a graduate student at the University of Washington’s School of Environmental and Forest Sciences. She studies the factors that influence how farmers are adapting to climate change and is especially interested in the role of seed diversity in climate resilience. She employs qualitative and quantitative methods that include conducting interviews, evaluating survey data, and analyzing satellite imagery. Her learning extends beyond the classroom and into the field as an avid gardener and part-time farmworker. Before graduate school, she did communications at FarmStand, a legal advocacy organization focused on building a more just food system. She graduated from Boston University in 2019 with a B.A. in International Relations and a minor in Biology.
The 2024-2025 seminars will be held in person, and are free and open to the public.