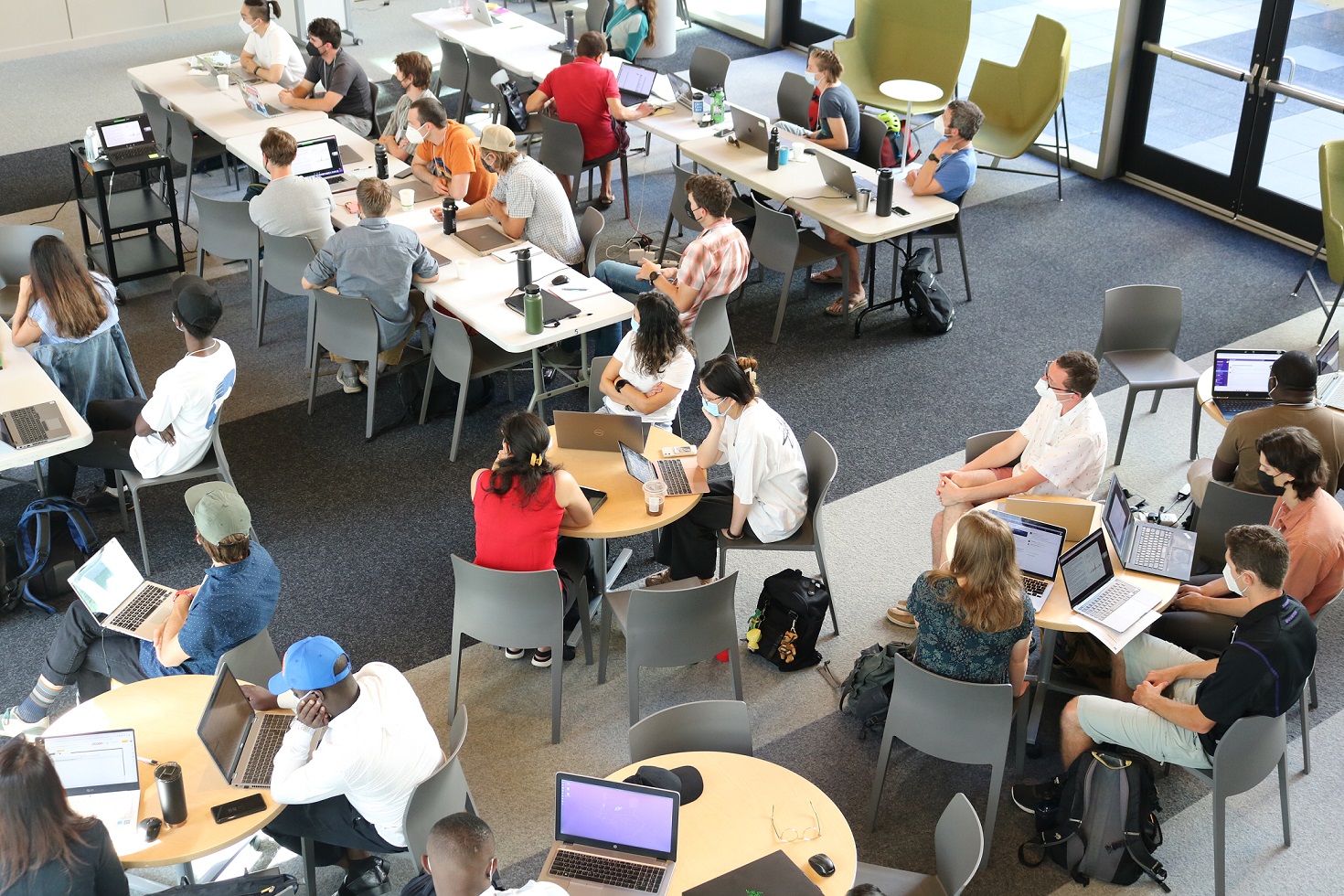
Advancing data-intensive discovery in all fields
Data science uses tools and techniques to make sense of complex datasets.
Happening Now
No Events
Sign Up for Data Science News
When you sign up for the eScience Institute’s email list, you’ll get the latest updates on upcoming data science news like events and programs, job opportunities, research features, and news from the UW and beyond.
Your personal information will only be used for eScience news and announcements, and will not be shared.
Special thanks to our sponsors: the University of Washington, the Gordon and Betty Moore Foundation, Schmidt Sciences, the Alfred P. Sloan Foundation, the Washington Research Foundation, the National Science Foundation, the National Institutes of Health, NASA, and the Department of Energy.